What is Metadata Analytics?
Metadata Analytics refers to the process of collecting, organizing, and analyzing metadata—the data that describes other data—to derive strategic insights, enhance data visibility, and fuel intelligent decision-making across the enterprise. It acts as a foundational pillar for modern data ecosystems, enabling organizations to decode the context, origin, structure, sensitivity, and lifecycle of data assets, without ever accessing the actual data itself.
In an era where 90% of enterprise data is unstructured and distributed across hybrid environments, Metadata Analytics provides the key to unlocking visibility, compliance, and control. It is no longer just a supporting function—it’s a core capability for driving AI readiness, zero-trust security, and regulatory alignment.
Why Metadata Analytics Matters in the AI-Driven Era
Enterprises are racing to operationalize AI and machine learning at scale, but there’s one catch: most AI models are being trained on chaotic, fragmented, and poorly understood data. According to IDC, by 2026, 80% of global enterprises will face significant delays in AI adoption due to ungoverned data environments and unclear data lineage.
That’s where Metadata Analytics becomes a game-changer.
It helps organizations answer mission-critical questions such as:
- Where does this data come from?
- Who has access to it—and should they?
- Is it compliant with regulatory frameworks like GDPR, HIPAA, or DPDP?
- Can this data be ethically used for AI training?
- Is this data duplicative, outdated, or high-risk?
Metadata analytics provides a 360-degree view of your data’s DNA—empowering smarter data governance, contextual risk management, and ethical AI development.
Key Components of Metadata Analytics
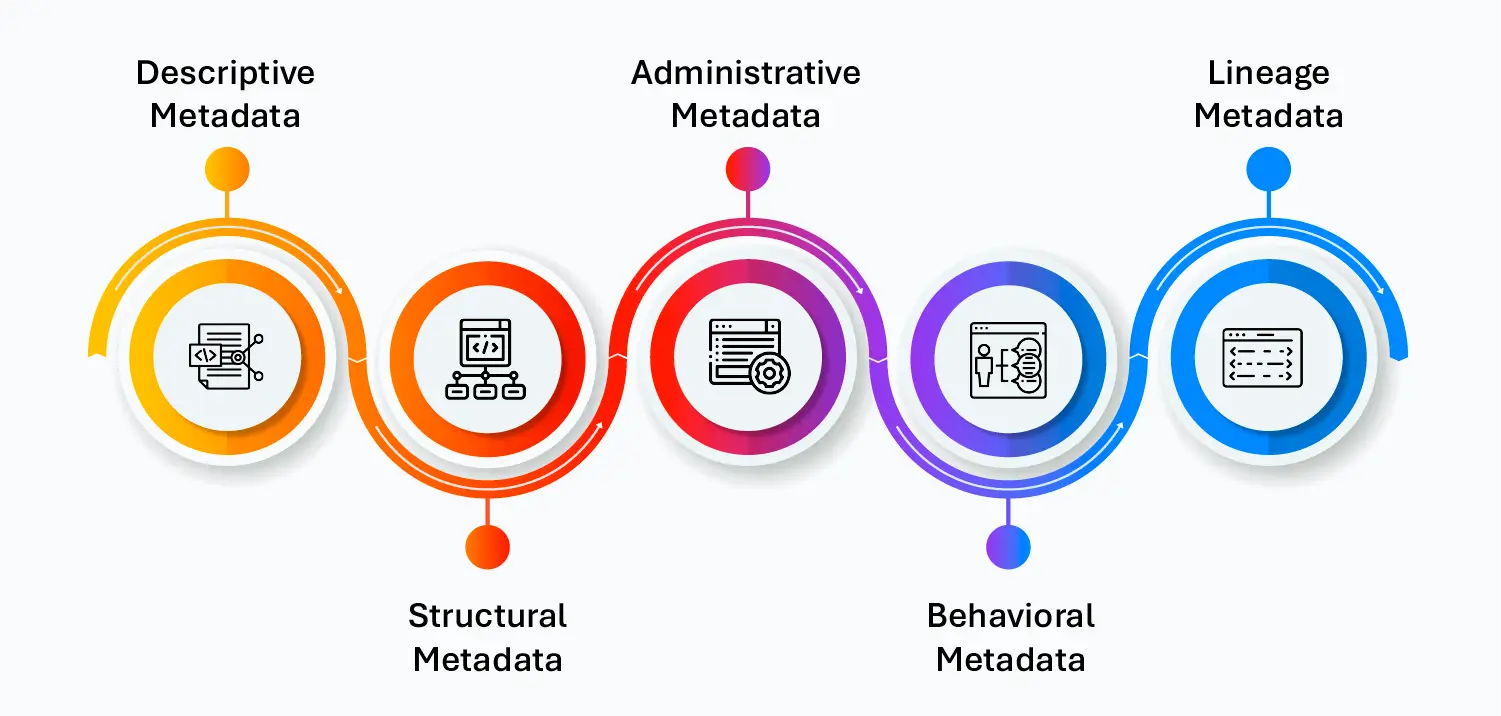
- Descriptive Metadata: Labels, titles, keywords, and tags that describe the content of data files. Enables efficient classification and discovery.
- Structural Metadata: Information about data models, schemas, formats, and relationships—essential for integrating datasets across hybrid or multi-cloud architectures.
- Administrative Metadata: Captures ownership, access permissions, modification timestamps, and retention policies—key for compliance and data security.
- Behavioral Metadata: Tracks user interactions with data—who accessed what, when, and how often. Crucial for usage auditing, access analytics, and insider threat detection.
- Lineage Metadata: Provides a traceable path of where data originated, how it has changed, and where it has moved. It’s the bedrock of data trust and transparency.
Benefits of Metadata Analytics
- Full-Spectrum Visibility: Discover and understand both structured and unstructured data across file shares, cloud object stores, emails, collaboration tools, and beyond.
- Actionable Intelligence: Move beyond data mapping to metadata-driven remediation—automate access controls, trigger policy workflows, and quarantine high-risk data.
- Ethical AI Enablement: Tag and filter sensitive data before it enters your model pipelines. Maintain accountability and consent compliance at every step.
- Operational Efficiency: Reduce infrastructure bloat by identifying ROT (Redundant, Obsolete, Trivial) data. Drive intelligent storage decisions across the hybrid cloud.
- Data Trust and Lineage: Build user confidence by surfacing the full history, context, and control layers behind every dataset.
Future Trends: Where Metadata Analytics is Heading
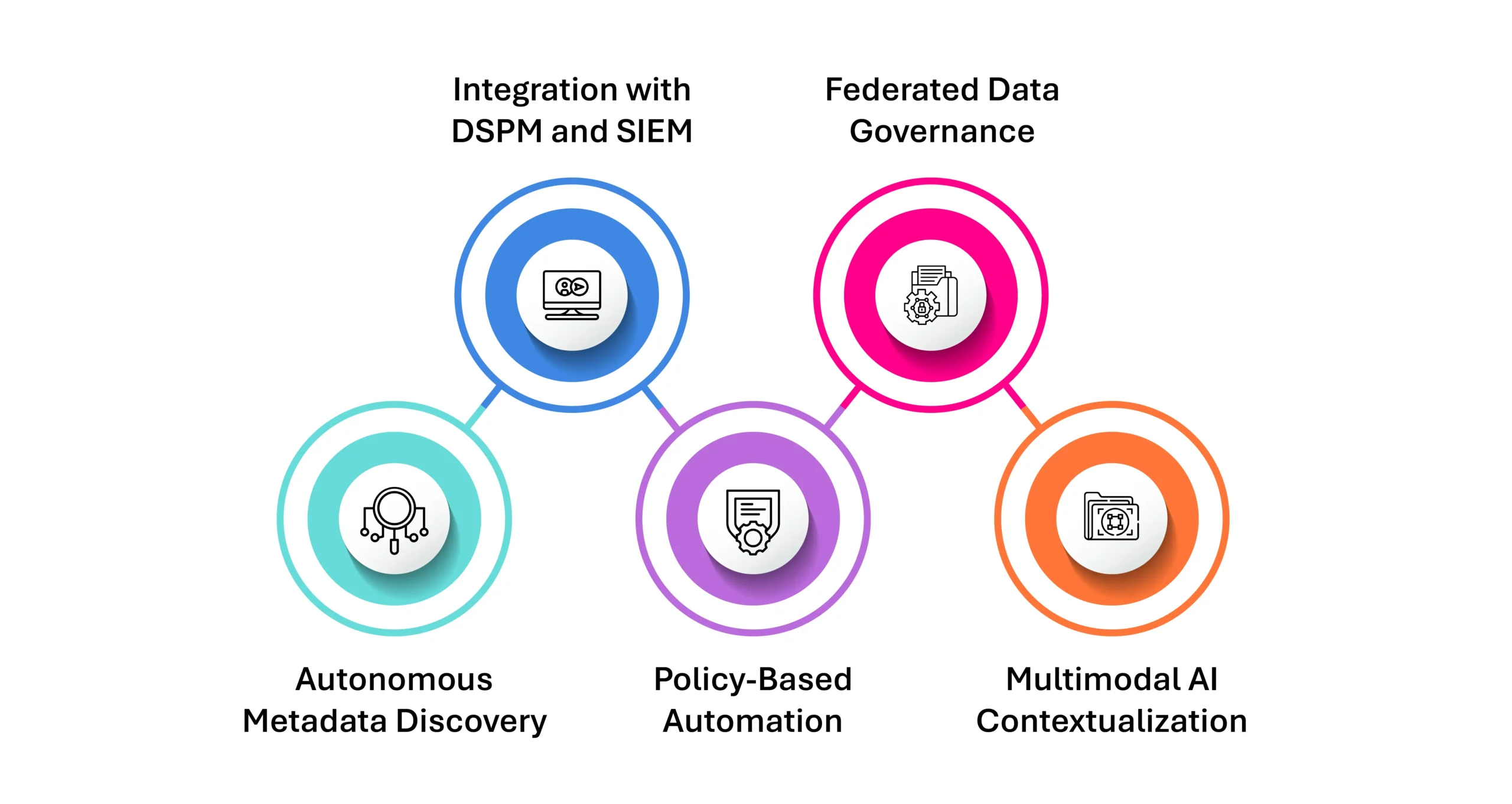
- Autonomous Metadata Discovery: AI-powered engines are replacing static scans with real-time, continuous metadata harvesting, improving accuracy and scalability.
- Integration with DSPM and SIEM: Metadata analytics is becoming a core input for Data Security Posture Management (DSPM) and security event platforms, closing the loop between visibility and action.
- Policy-Based Automation: Metadata will drive intelligent workflows, like auto-repermissioning files based on user roles, or triggering alerts when sensitive data is accessed by unauthorized users.
- Federated Data Governance: With decentralization on the rise, metadata analytics enables different data owners to govern their assets while maintaining a unified compliance backbone.
- Multimodal AI Contextualization: Metadata is being enriched with semantic context, allowing large language models (LLMs) to reason about data structure, sensitivity, and relevance before processing.
Metadata Analytics is no longer a backend function—it’s a strategic imperative. It empowers enterprises to tame the sprawl, democratize data access, embed compliance, and fuel innovation with trust. In a world where AI, privacy laws, and hybrid infrastructure are converging, metadata isn’t just describing your data—it’s defining your future.
Getting Started with Data Dynamics:
- Learn about Unstructured Data Management
- Schedule a demo with our team
- Read the latest blog: AI Is Only as Secure as the Data You Feed It. Is Yours Truly Ready?