In an era where businesses are racing to become data-driven organizations, the paradox is striking: despite having access to powerful cloud storage, scalable storage solutions, and advanced data modeling tools, many enterprises still struggle to derive value from their data. The culprit? Data silos—those quiet but persistent barriers that fragment insight, hinder agility, and compromise security and compliance.
In a landscape defined by real-time analytics, business intelligence, and heightened customer expectations, data silos are no longer just an IT headache. They’re a strategic liability.
Defining Data Silos in the Context of a Modern Enterprise
At its core, a data silo is a collection of structured data or unstructured information that’s isolated from the rest of the organization. It exists in a vacuum—often confined to a single team, application, or cloud storage environment—cut off from centralized data governance, integration workflows, and the broader data architecture.
And while silos may start innocently—a product team tracking user behavior in one app, while marketing tracks engagement in another—they quickly evolve into roadblocks that obstruct access to data, slow down innovation, and erode the foundations of data-driven decision making.
How Data Silos Undermine Strategic Business Goals
Modern enterprises thrive on data integration. They require seamless connectivity between data sources, real-time access control, and unified data quality standards to power everything from customer experience strategies to AI-driven operations. But when silos exist, those ambitions are compromised:
- Inconsistent insights: Business leaders receive conflicting reports because customer data is scattered across CRM systems, support platforms, and marketing tools.
- Redundant storage: Data is duplicated across silos, inflating storage solutions and cloud storage costs without adding value.
- Security vulnerabilities: Isolated systems often lack standardized security features, putting data security at risk and complicating regulatory audits.
- Delayed responses: Real-time responsiveness becomes near impossible when departments can’t collaborate using shared data views.
- Compliance nightmares: Meeting security and compliance mandates like GDPR or HIPAA becomes a Herculean task when data retention, access control, and audit trails vary across silos.
Simply put, data silos prevent organizations from unlocking the full potential of their data assets.
Why Do Data Silos Still Exist?
Despite massive investments in data management strategy, silos persist due to:
- Decentralized tech stacks: Teams adopting their own tools without considering enterprise-wide data architecture alignment.
- Poor data governance: Absence of overarching policies around data access, retention, and sharing protocols.
- Legacy systems: Older platforms that don’t integrate well with modern analytics or cloud storage environments.
- M&A activity: Acquiring companies with their own isolated data ecosystems and governance challenges.
These silos may not be intentional, but their consequences are undeniably strategic.
Breaking Down Data Silos: A Modern Blueprint
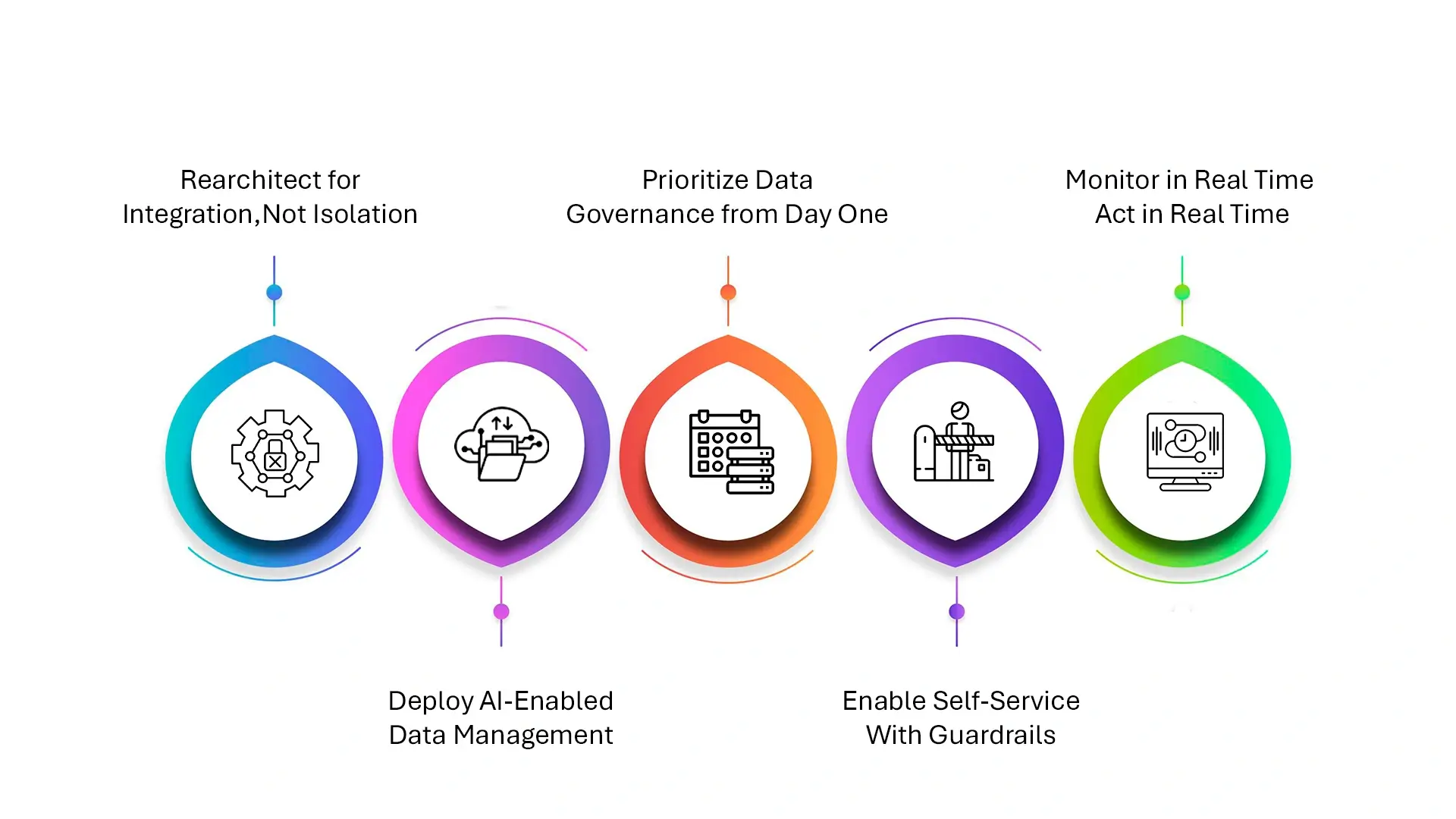
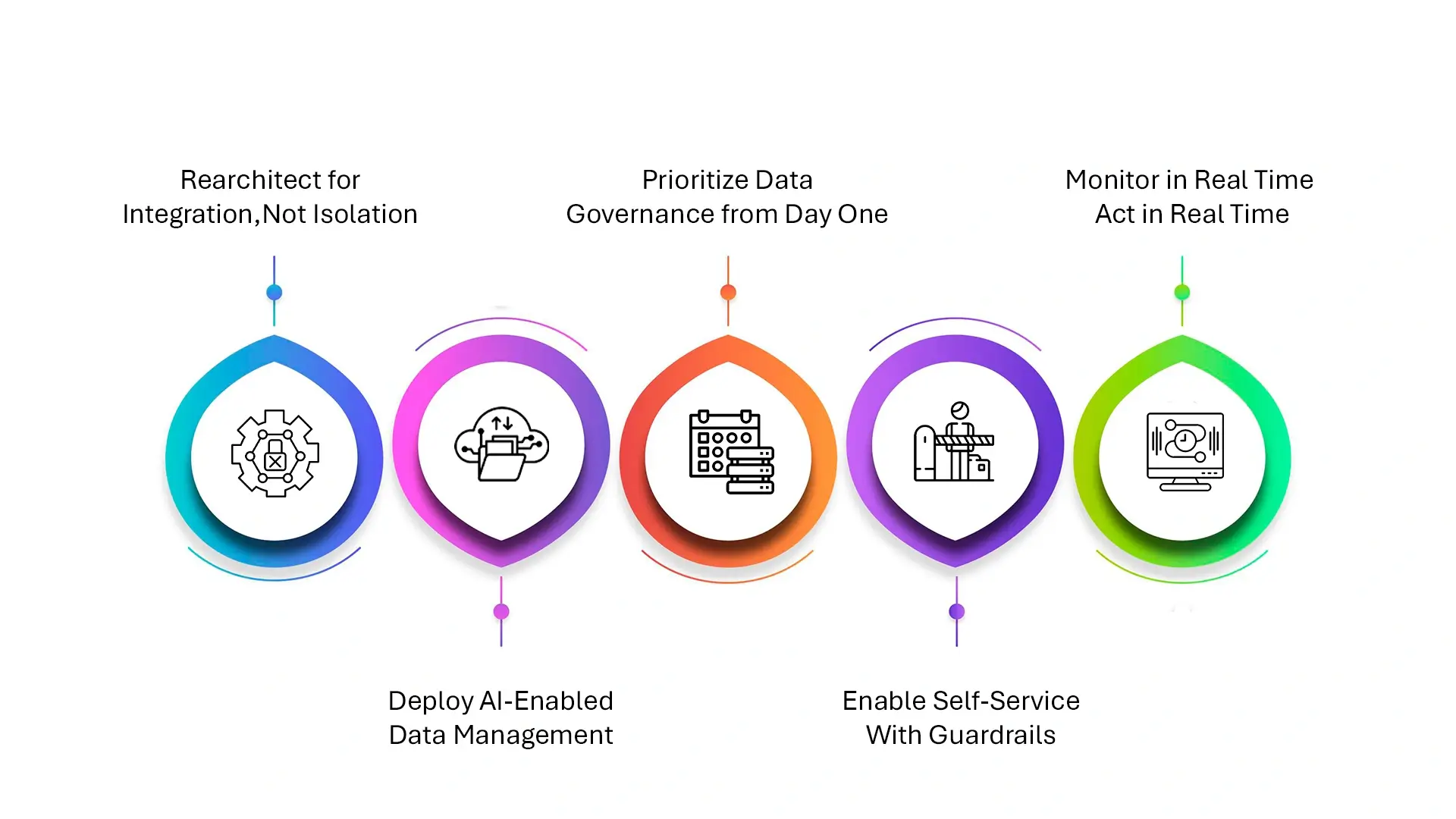
The road to eliminating data silos requires more than a tech fix—it demands a culture shift toward centralized data governance and federated control. Here’s how leading enterprises are tackling the problem:
1. Rearchitect for Integration, Not Isolation
A unified data architecture—ideally built on cloud-native principles—supports scalable, secure, and centralized data storage while allowing flexibility across departments. Embrace data fabric or data mesh models to enable localized ownership without fragmentation.
2. Deploy AI-Enabled Data Management
Advanced tools with embedded AI can identify redundant data sources, classify customer data, and automate policy-based data movement. These systems also enforce access control and optimize retention, enhancing data quality and compliance simultaneously.
3. Prioritize Data Governance from Day One
Building a data management strategy without governance is like constructing a skyscraper without a blueprint. Organizations must establish enterprise-wide policies for data integration, ownership, retention, and access to data—especially when operating in regulated sectors like finance or healthcare.
4. Enable Self-Service With Guardrails
Empower users to access and work with data in a controlled, compliant environment. Self-service analytics, layered with robust security features, accelerate productivity without exposing the business to risk.
5. Monitor in Real Time, Act in Real Time
Modern enterprises need to move beyond batch-based data management. Real-time dashboards, alerts, and anomaly detection tools ensure immediate visibility across silos, reducing downtime and enabling proactive governance.
Strategic Benefits of a Silo-Free Enterprise
Removing data silos doesn’t just improve operations—it elevates your entire business strategy:
- Better customer experience through unified customer data profiles and consistent interactions.
- Faster, smarter decisions powered by complete, real-time data.
- Stronger security posture, with centralized oversight of data security and retention policies.
- Reduced storage costs and operational inefficiencies.
- Future-proof compliance, aligning with data protection regulations and internal policy mandates.
In short, you don’t just become data-aware—you become data-intelligent.
From Fragmentation to Transformation
Today, data is the fuel behind every competitive advantage—from personalized user journeys to predictive analytics and AI automation. But none of it is possible when your insights are trapped in silos. Businesses that want to thrive in a data-driven economy must adopt a data management strategy that treats integration, governance, and accessibility as non-negotiables.
Data silos are not just technical flaws—they’re strategic risks. And the businesses that dismantle them now are the ones that will lead tomorrow.
Getting Started with Data Dynamics:
- Learn about Unstructured Data Management
- Schedule a demo with our team
- Read the latest blog: Smarter Data, Smarter AI: Why Your AI Strategy Hinges on Data Quality