What is Data Quality?
Data Quality refers to the measure of data’s condition based on attributes like accuracy, completeness, consistency, reliability, and validity. High-quality data faithfully represents real-world scenarios and is fit for its intended uses across operations, analytics, and strategic decision-making.
Why It Matters
In today’s data-centric world, poor data quality isn’t just a technical issue—it’s a business risk. From skewed insights and inefficiencies to reputational and regulatory fallout, the cost of unreliable data is significant. As AI and automation increasingly drive decision-making, the quality of input data directly shapes the outcome. Simply put, you can’t make intelligent decisions with unintelligent data.
Key Dimensions of Data Quality
- Accuracy: Reflects how well data mirrors the true value of what it represents.
- Completeness: Indicates whether all required data is available and captured.
- Consistency: Ensures data is coherent and harmonized across systems.
- Timeliness: Confirms that data is current and available when needed.
- Validity: Reflects adherence to rules, formats, or schemas.
- Uniqueness: Prevents redundant or duplicate records in datasets.
Challenges in Maintaining Data Quality
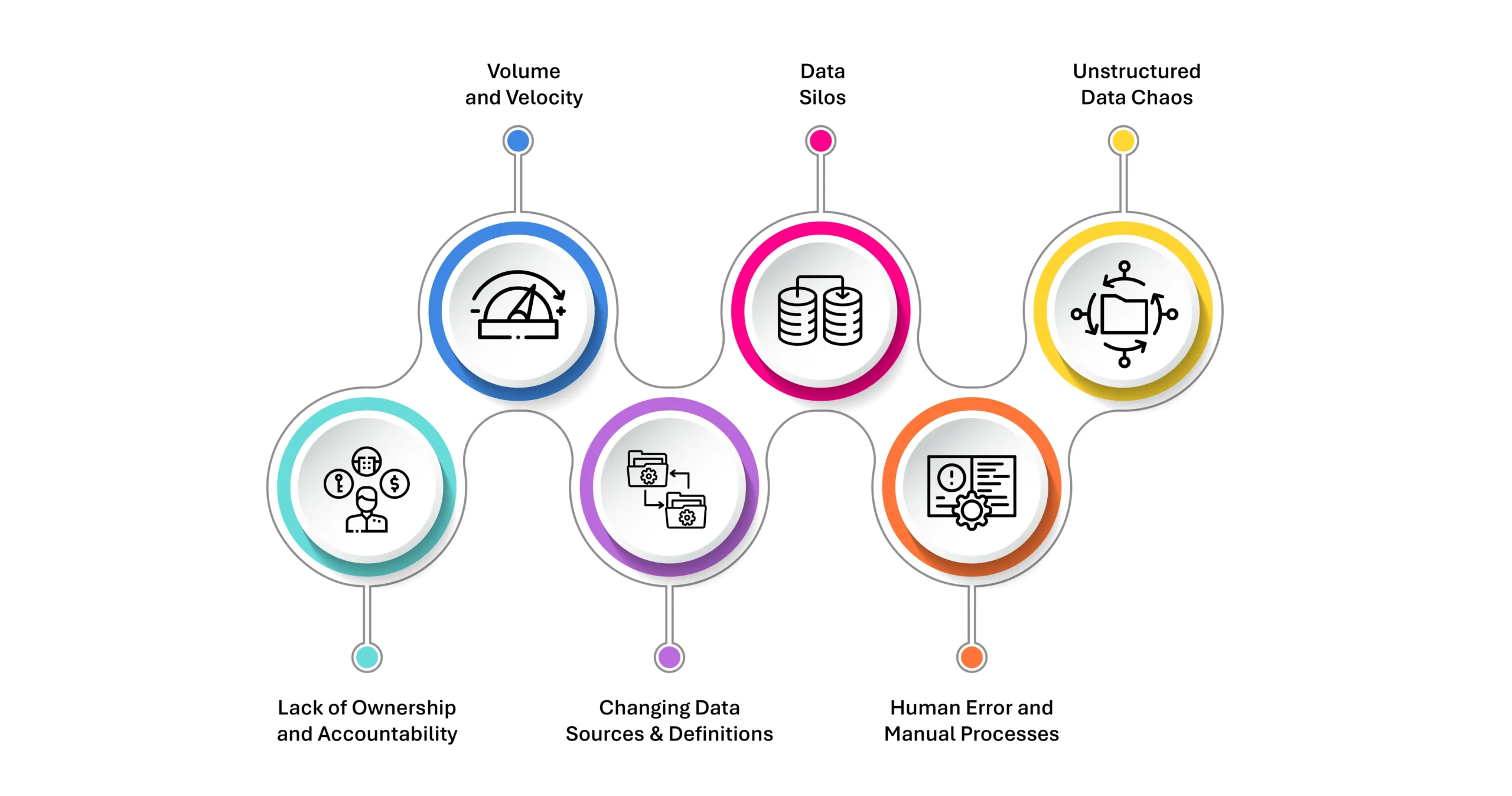
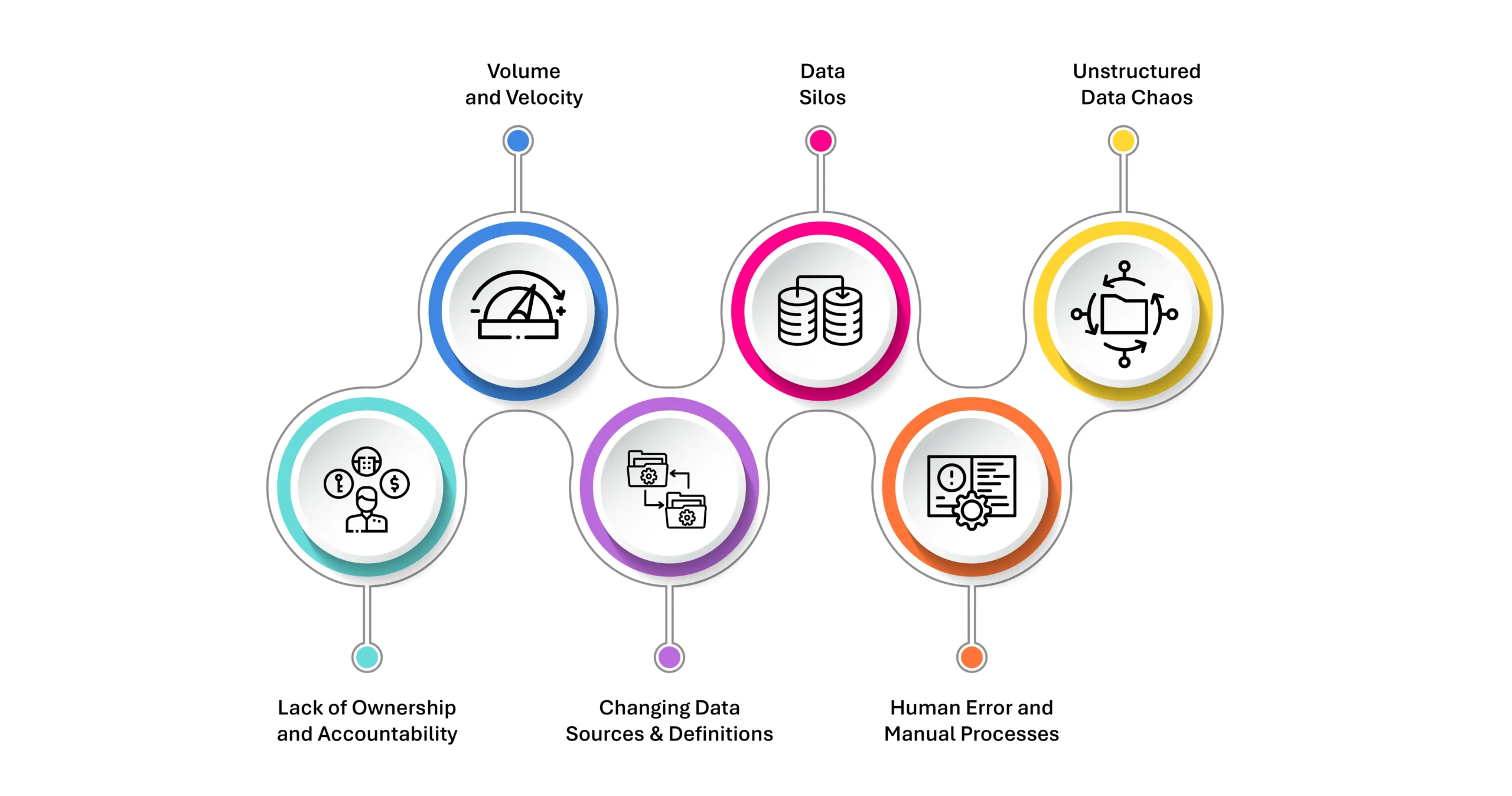
Data quality management is no longer a one-time initiative—it’s a continuous, enterprise-wide discipline. And it’s increasingly complex. Organizations are inundated with data from countless sources: legacy systems, cloud applications, IoT devices, customer touchpoints, and third-party platforms. This data explosion brings with it a set of evolving challenges:
- Volume and Velocity: The sheer scale and speed of incoming data can outpace traditional quality checks. High-volume streams require real-time validation and cleansing, which many legacy systems can’t handle.
- Data Silos: Different departments often operate in isolation, leading to fragmented and inconsistent data. Without a unified governance framework, reconciling these silos becomes a daunting task.
- Unstructured Data Chaos: Text documents, PDFs, images, and other unstructured formats now constitute over 80% of enterprise data. These are harder to validate and enrich due to their lack of predefined structure.
- Human Error and Manual Processes: Inaccurate data entry, poor tagging, and inconsistent naming conventions—especially in environments relying on manual data handling—can introduce significant quality gaps.
- Changing Data Sources and Definitions: As business needs evolve, so do the definitions of data fields and quality benchmarks. Without agility in data governance, organizations struggle to keep up.
- Lack of Ownership and Accountability: Data quality often falls between the cracks of IT and business teams, with no single point of ownership. Without clear accountability, quality initiatives stagnate.
The Role of Technology in Data Quality
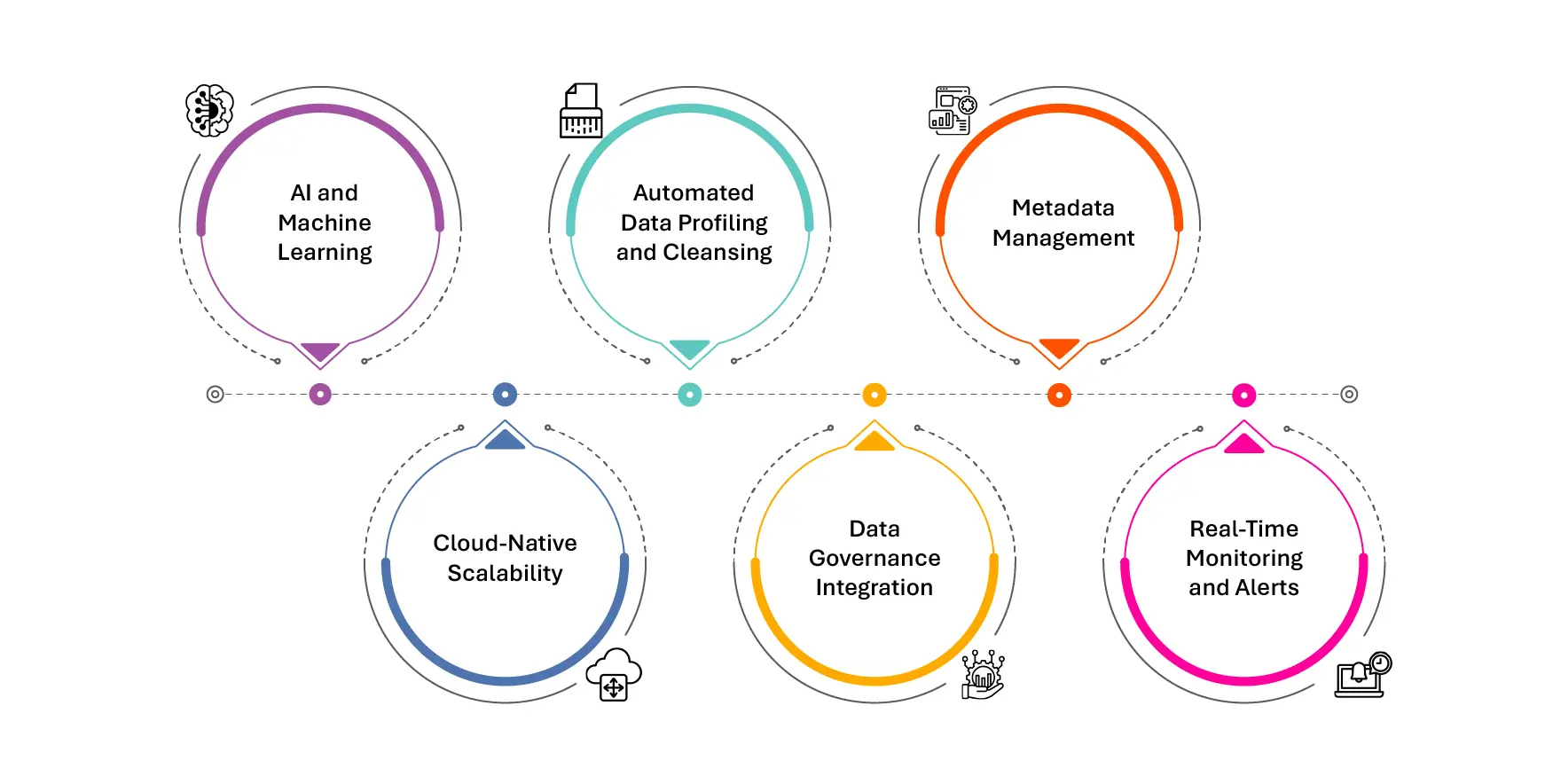
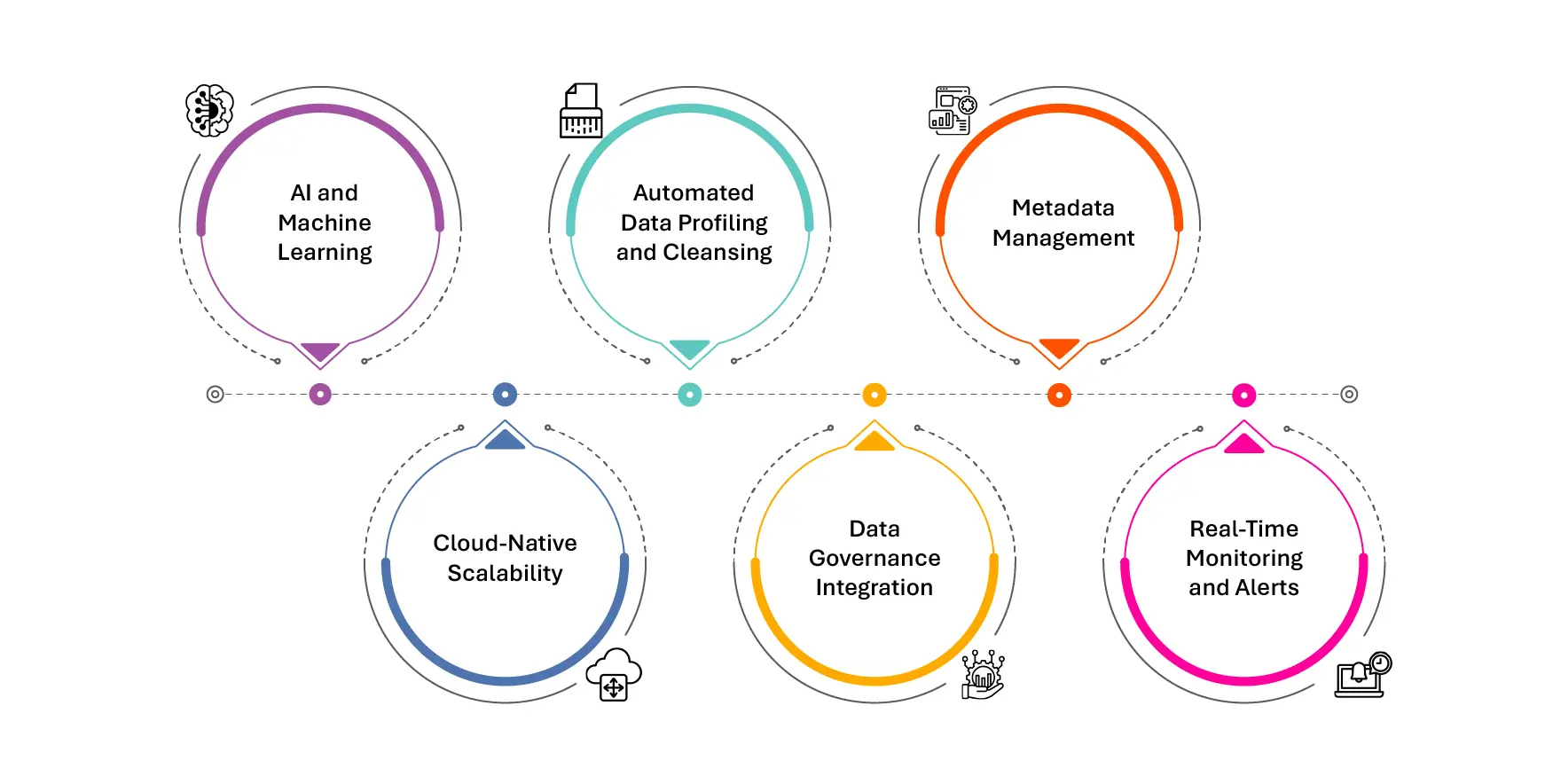
To meet the demands of real-time, high-stakes data environments, technology has become a cornerstone in the pursuit of quality. Modern solutions do more than just validate entries—they enable intelligent, automated, and scalable data quality management:
- AI and Machine Learning: Smart algorithms now identify anomalies, detect duplicate records, and suggest corrections without manual intervention. ML models can adapt to evolving data patterns, flagging inconsistencies that static rules would miss.
- Automated Data Profiling and Cleansing: Profiling tools scan datasets to uncover quality issues—null values, outliers, format violations—while cleansing engines standardize, enrich, and correct data at scale.
- Metadata Management: Advanced tools extract and interpret metadata, enabling a deeper understanding of data context and lineage, which is essential for resolving quality issues across systems.
- Real-Time Monitoring and Alerts: Dashboards and alert systems monitor data flows continuously, notifying teams of quality degradation the moment it happens. This reduces lag between detection and correction.
- Data Governance Integration: The best tools don’t operate in silos—they plug into broader governance frameworks, linking data quality policies with access controls, compliance mandates, and audit trails.
- Cloud-Native Scalability: Modern data quality platforms are built for the cloud, ensuring they can scale across hybrid and multi-cloud environments where data constantly moves and evolves.
High data quality ensures better AI model training, more reliable predictive analytics, smoother customer journeys, and ultimately, better business outcomes. As organizations embrace decentralized and self-service data access, ensuring the underlying data is clean, contextualized, and reliable becomes critical. Data quality, therefore, isn’t a checkbox—it’s a strategic imperative for digital transformation, ethical AI, and competitive advantage.
Getting Started with Data Dynamics:
- Learn about Unstructured Data Management
- Schedule a demo with our team
- Read the latest blog on Data Quality: Data Quality: A Persistent and Dynamic, Ever-Evolving Challenge, Not Just a One-Time Fix