In today’s fast-paced and data-driven world, the finance industry increasingly relies on data to make informed decisions. However, access to vast information does not always guarantee success. Without proper understanding of data and its management, businesses can suffer from analysis paralysis, rendering valuable resources useless that could have helped them grow exponentially. In this blog post, we will explore the concept of data literacy in finance and its importance in decision-making processes. Join us as we uncover the secrets of successful data management in the finance industry!
What is Data Literacy? Why is it Important to Understand the Data You Have?
The finance industry is facing a massive challenge: unstructured data sprawl. With the explosion of unstructured data like emails, social media, and digital documents, financial institutions are grappling to keep up with the sheer volume of information at their fingertips. The catch? This data is bursting with valuable insights, and failure to manage and analyze it correctly can lead to missed opportunities and increased risk.
It’s a race against time for finance organizations to find effective solutions that can tackle unstructured data sprawl. The urgency of the matter cannot be overstated, and it has become a top priority for many organizations in the industry. The question is, what should they do rise to the challenge and seize the untapped potential of unstructured data? The answer lies in ensuring Data Literacy. Before exploring the benefits of Data Literacy and its nuances, let’s take a look at some of the ongoing trends in the financial sector today, their implications and the solutions.
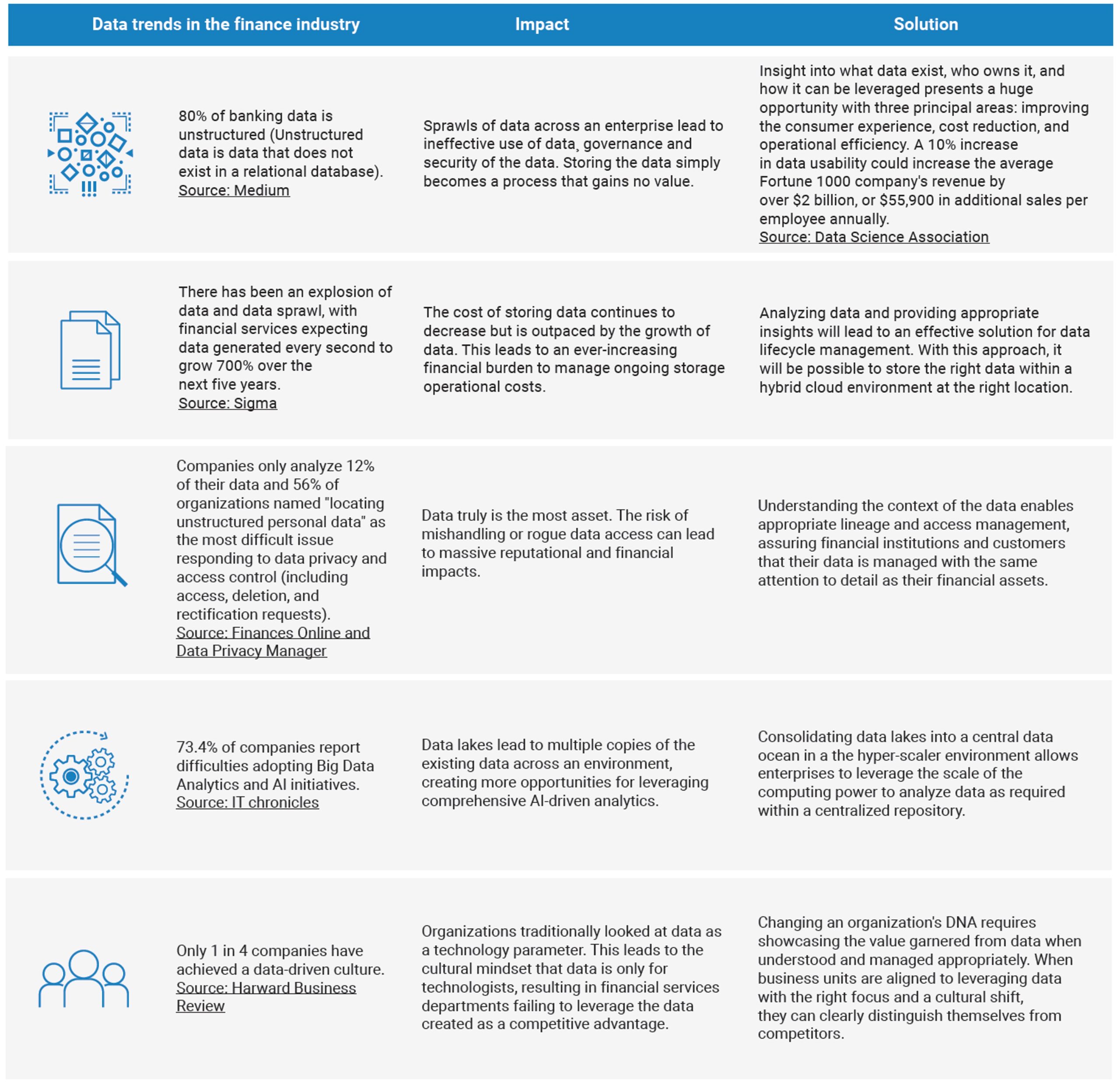
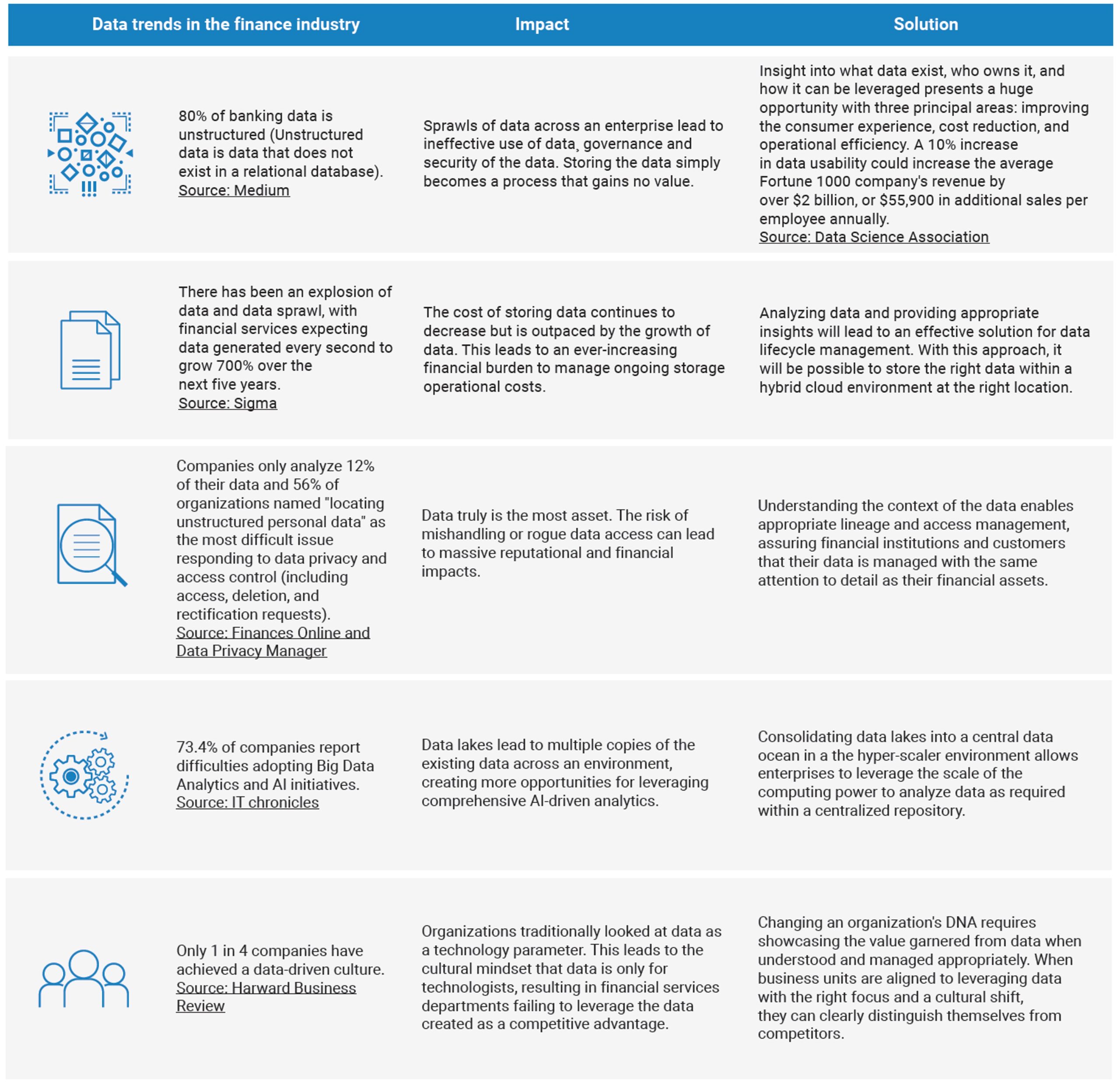
The key to unlocking all the solutions mentioned above begins with a simple but crucial step – understanding enterprise data. And that’s precisely where the power of data literacy comes into play.
It is the ability of individuals or organizations to read, analyze and interpret data. This includes understanding how to access, evaluate and use data effectively for decision-making purposes. In today’s world, where data is ubiquitous, mastering data management techniques has become a necessity for financial professionals. With AI and machine learning, finance companies are collecting more data than ever before, but without proper training, they risk being overwhelmed by its sheer volume. Data literacy involves not only understanding how to access and evaluate data but also recognizing its biases and limitations. It is the art of knowing which questions to ask and which datasets to use to find the answers. Data analysis skills help identify trends and patterns that can inform better business decisions. But to derive maximum value from data, individuals must also possess analytical and communication skills to share insights effectively.
To make sense of data, it’s essential to identify relevant data points and filter out irrelevant information. By doing so, we can focus our attention on what matters most and avoid getting lost in the noise. Additionally, understanding the limitations of data is crucial to ensure that our analysis is accurate. Data can be incomplete or biased, and being aware of these factors helps us mitigate risks associated with financial decision-making processes. Understanding how different pieces fit together gives us greater insight into complex problems, ultimately driving better investment outcomes.
Let’s start by asking ourselves the most basic question.
Do You Need All the Data You Have?
Data is the backbone of any finance-related decision-making. However, having too much data can be overwhelming and lead to analysis paralysis, where too much time is spent analyzing data without taking any action. According to Gartner, 80% of the data generated is dark, described as unutilized information because it’s often hidden away in various databases and systems, making it difficult to access and analyze. 91% of companies report that poor data wastes revenue and about 88% of data is ignored. Therefore, it is important to identify the relevant data points to help answer the question and filter out the noise. Here are some steps that can help:
- Identifying Relevant Data Points:
To identify relevant data points, it is essential to have a deep understanding of the business and the industry. This involves knowing what data is essential for decision-making and what is not. For example, if you are trying to decide whether to invest in a company, you may want to look at financial data such as revenue growth, profitability, and debt levels.
- Filtering Out the Noise:
Filtering out the noise involves getting rid of data that is not relevant to the decision at hand. It is essential to focus on accurate, up-to-date, and actionable data. For example, if you are trying to determine the effectiveness of a marketing campaign, you may want to focus on metrics such as click-through rates, conversion rates, and return on investment. Data that does not directly relate to these metrics, such as website traffic, may not be relevant and should be filtered out. Effective filtering techniques require understanding your business objectives and how best to analyze available data sources without getting lost in irrelevancies – this requires practice and attention!
- Understanding the Limitations of Data:
Data can be biased, incomplete, or inaccurate, and it is important to be aware of these limitations when making decisions based on data. This requires critical thinking and questioning of the data and its sources. For example, financial data may be influenced by accounting methods or the industry standards used. Therefore, it is crucial to understand the context in which the data was collected and analyzed. Understanding these limitations will help prevent over-reliance on flawed assumptions based on incomplete datasets, thereby leading you toward accurate analysis and decision-making with available financial resources.
The Importance of Data Literacy in Finance
Data literacy has become a game-changer in finance, empowering experts to analyze and interpret financial data with unmatched precision. The financial sector relies heavily on data to gauge performance, detect trends, and make informed decisions, and data literacy is the ultimate weapon for achieving that.
The ability to access, understand, and derive maximum insight from data sprawls, regardless of technical proficiency, helps individuals across the breadth of an organization make sense of complex data and make informed decisions. Imagine if this would happen – every single employee would be armed with the ability to innovate and generate value based on statistical insights. No obstacles, no hesitation, just a seamless flow of efficiency. It’s like a dream come true!
Data literacy is also the ultimate security and compliance tool in today’s constantly evolving regulatory environment. It’s not just about extracting valuable insights from financial statements, market data, or customer data, but about being able to mitigate risks and identify game-changing opportunities that could shape the future of your business.
When you have a solid understanding of your data, you’ll know precisely what lies within it and how best to protect it. In addition, with the finance sector regulations becoming increasingly complex and demanding, the ability to analyze and interpret data accurately has never been more crucial. Without it, you run the risk of incurring heavy penalties and fines.
Lastly, data literacy is the bridge between innovation and success in the finance sector. In a sector that is continually evolving, innovative solutions are necessary to meet customer needs. Experts in finance need to use data to identify new opportunities and develop cutting-edge solutions that set them apart from their competitors.
To sum up, data literacy is the bedrock of success in finance. However, with an excess of data at our fingertips, it can become a liability if not managed correctly. Therefore, finance professionals must possess strong critical thinking abilities to interpret information accurately. Simply having access to a wealth of data is not enough if its relevance or limitations are not understood, and this can lead to analysis paralysis, stunting growth and progress.
The Danger of Analysis Paralysis
One of the biggest risks associated with data literacy in finance is analysis paralysis. This occurs when individuals become so overwhelmed by the amount of data available that they can’t make any decisions. Instead, they get stuck in a loop of analyzing and re-analyzing the data without progressing. The crux of the challenge lies in unstructured data. This is like a black hole of information with no one knowing anything about it. It’s just an enormous sprawl of sensitive, cold, hot, and ROT data (redundant, obsolete, and trivial) taking up space, cost and time.
Analysis paralysis can particularly damage finance, leading to missed opportunities or poor decision-making. For example, if an investment company spends too much time analyzing different stocks and funds, it may miss out on profitable investments. Furthermore, this state of over-analysis can also lead to decision fatigue which often happens when people are forced to make too many choices as part of their job. When you’re dealing with such an enormous amount of information every day, there comes a point where your mind simply cannot process it anymore. To avoid falling into analysis paralysis, it’s important to set clear goals for what you want to achieve from your data analysis efforts. Moreover, you need not over-analyze irrelevant details but focus only on relevant factors to help you reach your goals faster and more effectively. While financial professionals need to have strong analytical skills and be able to interpret complex datasets accurately – getting caught up in endless layers of detail is counterproductive. By focusing only on relevant information needed for optimal results, one can avoid costly mistakes stemming from analysis paralysis syndrome! Enterprises need to create data-powered financial services ecosystem based on intelligent data management. The way businesses interact with their clients is fundamentally altered because of data-powered banking. Nowadays, companies can answer questions related to customer behavior and everyday business operations within seconds instead of days and, at times, years. As a result, several financial institutions have seen an increase in efficiency and a decrease in expenses.
The Role of Critical Thinking in Data Analysis
In the finance industry, data analysis is a crucial part of decision-making. However, it’s not just about having access to the data or knowing how to use analytical tools; critical thinking is important in ensuring that the insights derived from data are accurate and useful. Critical thinking involves evaluating information objectively based on relevance, accuracy, completeness, and reliability. In other words, it means considering all possible factors before concluding. When applied in data analysis, critical thinking helps prevent errors due to biases or incomplete information by challenging assumptions and verifying results through independent research or consultation with experts.
Having strong critical thinking skills also enables professionals in finance to identify patterns and trends that might be missed by relying solely on automated processes or standard procedures. This allows them to make more informed decisions that consider both quantitative and qualitative aspects of a problem.
Developing strong critical thinking skills is essential for anyone in finance who wants to leverage data effectively. By using logic and reasoning to evaluate information systematically, individuals can make sound decisions based on reliable insights from their analyses.
But first start by inculcating a data literate culture. Empower employees to know their enterprise data – What and where is the data? Who has access? Is it secured and compliant?
Boosting Data Literacy: The Three Essential Building Blocks
The first and foremost barrier to ensuring Data Literacy is Unstructured data, i.e. data that lacks a defined structure or format. It can be serious roadblock and have grave consequences. Firstly, it’s like searching for a needle in a haystack – difficult to find, categorize, and organize. Without the right tools and skills, extracting insights from unstructured data can be a real challenge. Secondly, unstructured data can be a bit of a puzzle, lacking a clear structure or schema. This can leave users scratching their heads, trying to figure out how all the pieces fit together. Without a clear understanding of the data’s structure, users may struggle to interpret the data correctly and draw accurate conclusions. Thirdly, analyzing and visualizing this kind of data can be like trying to climb a mountain – especially when dealing with large volumes. Without the right data analysis and visualization skills, users may struggle to make sense of complex data sets and miss out on important insights. Finally, it can be a bit of a wild card, prone to errors and inconsistencies. This can shake users’ confidence in the data and limit its usefulness. However, with the right tools and techniques, users can conquer unstructured data and unlock its full potential to drive insights and informed decision-making. Here are three tools that can be used to limit the impact of unstructured data, as explained below:
Metadata analytics
Metadata analytics can play a significant role in improving data literacy by enabling users to find, understand, and use data more effectively. Here are some ways metadata analytics can enhance data literacy:
- Classify and categorize data, making it easier for users to find the information they need: By tagging data with relevant metadata, such as keywords, topics, and themes, users can quickly locate and access the data they require. This can help to reduce the time and effort required to find and use data, improving data literacy.
- Understand the characteristics of data: Gaining insights about the format, structure, and location of data, can help users to identify relevant data for their needs and understand how to use it effectively. By analyzing metadata associated with data, users can gain valuable insights into the nature of the data, which can help to improve data literacy.
- Identify relationships between data sets, making it easier for users to draw insights and make informed decisions: By analyzing metadata such as data lineage and data relationships, users can understand how different data sets relate to each other and identify opportunities for analysis and decision-making.
- Access only relevant data: By categorizing and tagging data as hot, cold, or ROT (redundant, outdated, or trivial), organizations can take appropriate actions such as archiving or deleting data and keeping only the necessary information for analysis and insights.
For more insights, check out Data Dynamics Metadata Analytics.
Content analytics
Content analytics can play a crucial role in increasing data literacy by providing insights into the content of unstructured data. Unlike metadata analytics, which focus on the descriptive information about the data, content analytics aim to understand the meaning and context of the data itself.
- Identify relevant data and extract insights: By using natural language processing (NLP) and machine learning techniques, content analytics can automatically classify, extract, and summarize key information from unstructured data. This can help users to quickly identify relevant data and gain a deeper understanding of its content.
- Visualize and explore their unstructured data in new ways: Using techniques such as topic modeling and sentiment analysis, can identify patterns, trends, and relationships in the data. This can help users to discover new insights and generate hypotheses that they may not have considered before.
- Locate and label business sensitive information that is hidden within unstructured data sprawls: By scanning through the content of unstructured data files, content analytics can identify sensitive or private data with a high degree of accuracy, including personally identifiable information (PII) and protected health information (PHI). Additionally, many software tools use advanced logical expressions and operators to generate actionable insights into risk exposure through descriptive and diagnostic analytics. Content analytics also offers capabilities for proactive risk mitigation and enables scalable data security remediation, such as moving high-risk personal or sensitive files to more secure storage, quarantining sensitive data and levy appropriate access controls. This empowers data custodians to secure their company’s data, comply with security regulations, and embrace data-driven management strategies.
- Improve the accuracy and quality of their data: Entity recognition and text normalization can ensure that data is consistent and standardized, reducing the risk of errors and misunderstandings. This can improve data literacy by providing users with a more reliable and trustworthy source of information.
Data Archival
Data archival is like storing your old clothes in a separate closet, away from your everyday outfits. It’s a process of identifying and transferring inactive or rarely accessed data to a secure and cost-effective storage system for long-term retention. This ensures that the valuable data is preserved for future reference, compliance, or legal requirements. Archiving is done by moving data to different types of storage systems like tape, disk, cloud, or object storage. By freeing up space in primary storage systems, it improves system performance, reduces costs, and allows organizations to prioritize their data and focus on the most important and relevant information.
- Access to important data: By archiving data, organizations can keep their active data sets leaner, which makes it easier for users to find and analyze the important data they need. This can help to improve data literacy by reducing the amount of time and effort needed to find and understand data.
- Data organization: Organizations can organize their data in a more structured way, making it easier to find and understand. This can be achieved through the use of metadata, which can be used to tag and categorize archived data, making it easier to search and analyze.
- Data retention policies: Data archival according to specific retention policies, organizations can ensure that important data is kept for the appropriate length of time. This can help to improve data literacy by ensuring that users have access to relevant historical data when needed, enabling them to make better-informed decisions.
- Compliance: Retaining data for the required length of time, and being able to produce it when required, ensures higher adhering to the relevant regulations and laws. This can help to improve data literacy by ensuring that users are aware of the regulations and requirements that govern their data use.
The Data Dynamics Advantage
In today’s fast-paced and data-driven world, organizations are collecting and managing more information than ever before. But with data scattered across multiple systems and departments, it can be overwhelming to make sense of it all. That’s where unified data management comes in. By consolidating all data into one platform and implementing standardized processes, enterprises can revolutionize their approach to data management and ensure data literacy across the organization.
Data Dynamics has been working with global giants for over a decade now to help them establish a data literate culture. The company’s goal is to empower enterprises to achieve data democratization, allowing users of all technical backgrounds to instantly access, understand, and derive maximum insights from unstructured data sprawls using its unified unstructured data management platform. The platform has already gained the trust of over 28 Fortune 100 organizations and includes four modules – Data Analytics, Mobility, Security, and Compliance – all within a single software. Utilizing a mix of automation, AI, ML, and blockchain technologies, it scales to meet the requirements of global enterprise workloads. With Data Dynamics, enterprises can analyze and migrate data based on their needs and the importance of the data. Enterprises can utilize one software platform to structure their unstructured data, unlock data-driven insights, secure data, ensure compliance and governance, and drive cloud data management.
To discover more about how Data Dynamics can help you streamline your data management, please visit www.datadynamicsinc.com or contact us at solutions@datdyn.com / (713)-491-4298 / +44-(20)-45520800.