As artificial intelligence accelerates its transformation of enterprise value chains, the real question for leaders is no longer “Do we need AI?” but rather, “Is our organization’s data truly ready for it?”
By 2027, global spending on AI systems is projected to surpass $500 billion, with enterprise investments pouring into cognitive software, next-gen infrastructure, and AI-centric operations (IDC). But beneath the surface of this spending surge lies a harder question for CIOs and CISOs alike:
Is your data infrastructure truly prepared to fuel AI? Or are you running multimillion-dollar algorithms on incomplete, unsecured, and context-starved data sets?
For forward-looking enterprises, data readiness—not just AI adoption—is the real differentiator. And unstructured data is where that readiness will be won or lost.
Why Unstructured Data is the Make-or-Break Factor for AI
By the end of 2025, 80 – 90% of all data generated will be unstructured, according to IDC. Yet most AI pipelines today are still optimized for structured databases—leaving vast quantities of emails, PDFs, radiology images, scanned contracts, call logs, and IoT feeds locked outside the AI value chain.
In fact, McKinsey notes that enterprises using high-quality, holistic data sources see up to 30% greater productivity from AI initiatives than those relying on fragmented or siloed data.
The Risks of Ignoring the 90%:
- Operational Blind Spots: AI tools trained without context-rich unstructured data can miss nuanced insights, leading to suboptimal outcomes—especially in high-stakes sectors like healthcare and finance.
- Compliance Failures: As global regulatory scrutiny increases (EU AI Act, India’s DPDP Act, CCPA, GDPR), ungoverned data becomes a legal liability.
- Cybersecurity Exposure: Unstructured data often lives outside core governance frameworks, creating attack surfaces that are invisible to traditional controls. Over-permissioned file shares and orphaned data repositories are the top culprits in breaches.
- AI Bias & Hallucinations: Training AI models on partial truths leads to systemic errors, skewed outputs, and a loss of trust in mission-critical decision-making.
Take the healthcare sector. Doctors might rely on diagnostic AI to flag potential patient risks. But if that AI isn’t trained on diverse datasets—including medical notes, radiology images, or handwritten intake forms—it might miss early warning signs. That’s not just an operational miss; it’s a life-or-death one.
In finance, ignoring unstructured data like voice logs or internal memos can mean missing fraudulent behaviour or market shifts. In fact, the 2023 collapse of FTX highlighted the dangers of opaque, ungoverned data trails, much of which resided outside structured systems.
But therein lies the opportunity. The enterprises that crack the unstructured data code now won’t just be AI-ready—they’ll position themselves at the forefront of innovation, regulatory resilience, and data-driven decision-making.
A New Blueprint: The Self-Optimizing Data Ecosystem
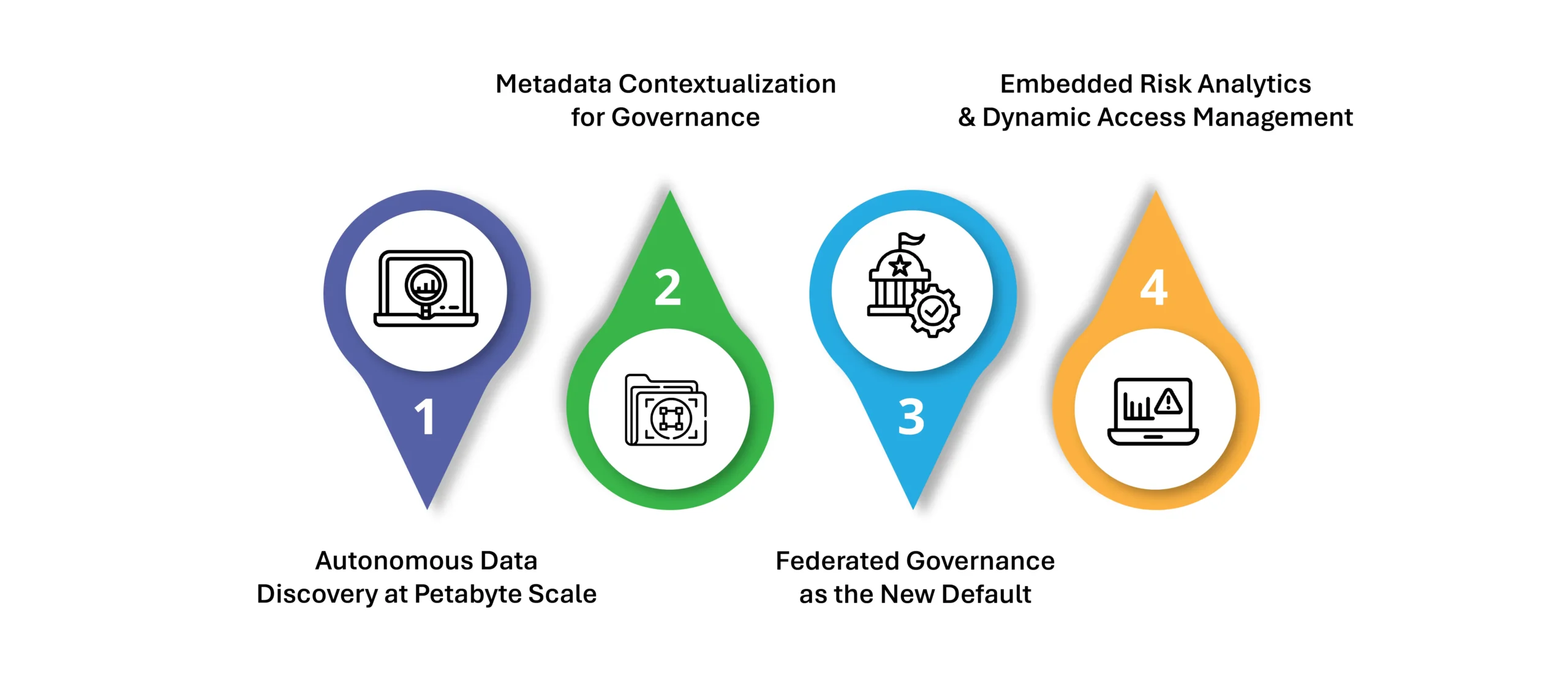
Leading CIOs and CDOs are coalescing around a new architecture—a self-optimizing data ecosystem. This isn’t just a storage strategy. It’s a dynamic, intelligent framework where data—structured and unstructured—is discovered, contextualized, governed, and secured in real time.
Built on a foundation of AI, automation, and decentralized control, this ecosystem is becoming the bedrock of enterprise resilience and innovation. Here’s what defines it:
- Autonomous Data Discovery at Petabyte Scale: Unstructured data can’t be secured or optimized if you don’t know it exists. Autonomous discovery engines powered by AI and machine learning are now scanning enterprise environments—cloud buckets, collaboration tools, email archives, and file shares—classifying data in real time based on sensitivity, usage patterns, and business relevance. Data Dynamics’ technology, for example, performs policy-driven discovery across hybrid cloud environments, giving organizations the visibility to identify ROT (redundant, obsolete, trivial) data, dark data, and high-risk assets.
- Metadata Contextualization for Governance: Unstructured data without metadata is a black hole—massive, invisible, and pulling risk into its orbit. The modern data stack now requires automated metadata enrichment at scale. Business-relevant metadata—such as data owner, regulatory classification, access history, and sensitivity score—is no longer a nice-to-have but a core pillar of compliance and AI modelling accuracy. Metadata engine continuously contextualize billions of files, enabling policy enforcement, audit trails, and intelligent automation.
- Federated Governance as the New Default: The centralized governance model is cracking under the weight of multi-cloud, multi-jurisdictional data sprawl. A federated model—where data and application owners manage policy execution within their domains—is emerging as the only viable path. With sovereignty laws tightening from the EU to the GCC, enterprises need systems that embed compliance into data workflows, not bolt it on afterward. This can be enabled by role-based, policy-driven governance at the edge of the data, ensuring decentralized control with centralized oversight.
- Embedded Risk Analytics and Dynamic Access Management: Zero Trust is no longer a security framework—it’s a survival mechanism. But trust can’t be zero unless visibility is total. The biggest threats today stem from shadow access, permission sprawl, and misaligned entitlements, especially across unstructured data assets. The future demands dynamic access controls that respond to behavioral signals, real-time risk scores, and regulatory requirements. Data Dynamics empowers this by embedding risk analytics, access remediation, and automated re-permissioning into the data fabric. This means that permissions adapt not just to roles, but to context—ensuring only the right people, at the right time, can access the right data.
Why This Strategy Pays Off
Let’s look at what a self-optimizing data ecosystem actually enables:
- Data for AI that’s Clean, Contextual, and Complete
AI models built on rich, contextualized unstructured data can power hyper-personalized customer experiences, nuanced diagnostics, or real-time fraud detection with unprecedented accuracy.
- Compliance that’s Proactive, Not Reactive
Instead of scrambling before every audit, enterprises can prove data lineage, permission histories, and access controls across every data point—structured or otherwise. This is crucial with regulations like the EU AI Act or India’s DPDP Act becoming enforcement ready.
- Sustainability Through Data Optimization
Storing unused data in triplicate across clouds isn’t just expensive—it’s environmentally unsustainable. Organizations that reduce data duplication, archive intelligently, and delete ROT (redundant, obsolete, trivial) data can reduce storage costs by up to 40%, and align with ESG goals.
- Faster Innovation Cycles
With AI-ready data available on demand, business units can prototype faster, model quicker, and scale smarter. Time-to-insight becomes time-to-advantage.
From Catch-Up to Catalyst
As we enter an AI-powered decade, the enterprises that will lead aren’t those with the most algorithms—they’re the ones with the most intelligent, agile data foundations beneath them. For CIOs and CISOs, 2025 is no longer about simply enabling AI adoption; it’s about engineering true AI readiness—particularly at the unstructured data layer, where chaos often eclipses clarity and opportunity.
The transformation is nothing short of tectonic. We’re evolving from rigid, siloed data warehouses to dynamic, self-optimizing ecosystems—where discovery is autonomous, governance is federated, risk is adaptive, and compliance is seamlessly embedded into every data workflow.
This is exactly where Zubin, Data Dynamics’ AI-powered self-service data management software, changes the game. Designed to tame unstructured sprawl at enterprise scale, Zubin turns fragmented data into a clean, contextual, and compliant asset—fueling smarter AI outcomes. With intelligent discovery, embedded risk analytics, and policy-driven governance woven into the fabric of enterprise infrastructure, Zubin doesn’t just help organizations prepare for AI—it enables them to lead the charge.