Highlights:
- In the midst of the financial transformation, Generative AI stands as the harbinger of change. Beyond data processing, this transformative technology is exploring novel frontiers such as predicting market sentiments, fortifying data security against quantum threats, and democratizing access to financial services with redefined credit scoring.
- Leading financial giants are harnessing the potential of Generative AI to improve risk management, enhance fraud detection, and streamline customer service, a testament to its tangible impact on daily operations.
- Effective data management is the secret sauce behind Generative AI’s success. It enables data integration, quality control, security, context awareness, advanced analytics, and real-time decision-making, resulting in robust risk assessments and impeccable customer satisfaction. It’s the cornerstone of innovation in the financial sector.
In this Blog:
The financial landscape is undergoing a profound transformation, and at the heart of this revolution lies the omnipotent force of Artificial Intelligence (AI). In recent years, AI has permeated every facet of the finance sector, reshaping the industry’s fundamental practices and unlocking new realms of possibility. In this age of digital disruption, one particular AI subset, Generative AI, has emerged as a game-changer, propelling the finance industry into uncharted territories of innovation. Its applications are permeating the core of financial operations, pushing the boundaries of what’s possible in this dynamic, data-rich, and fast-evolving landscape. In this article, we’ll delve into the world of Generative AI, exploring its neural networks, recurrent models, and its groundbreaking applications, all of which are reshaping the financial industry in profound ways.
Decoding Generative AI
Generative Artificial Intelligence, often referred to as Generative AI, is a fascinating subset of AI that goes beyond merely processing data and delves into the realm of content creation. At its core, Generative AI employs a combination of advanced neural networks and cutting-edge algorithms to understand and replicate intricate patterns, enabling it to craft content ranging from text and images to videos. This ability to generate content resembling human-produced output is a game-changer in the BFSI sector.
- Neural Networks and Generative AI
At the heart of Generative AI lies the neural network architecture, more specifically, deep neural networks. These networks are designed to emulate the human brain’s intricate structure, composed of interconnected artificial neurons. These neurons communicate with each other, enabling the network to process complex data and recognize patterns.
- Recurrent Neural Networks (RNNs)
In the context of text generation, Recurrent Neural Networks (RNNs) are pivotal. RNNs are characterized by their recurrent connections, allowing them to process sequential data effectively. This means they can capture contextual information, a crucial factor in generating coherent and contextually relevant text, which is particularly vital in BFSI for tasks like customer communication, financial report generation, and automated customer support.
- Variational Autoencoders (VAEs)
For tasks like image generation, Variational Autoencoders (VAEs) come into play. VAEs are adept at capturing the underlying structure and distribution of data. In the BFSI sector, VAEs can be employed for tasks like generating synthetic images for identity verification or creating visual representations of data, making complex financial information more accessible.
- GANs
Generative Adversarial Networks (GANs) are arguably the driving force behind Generative AI’s potential. GANs consist of two networks – a generator and a discriminator – that work in tandem. The generator aims to create content while the discriminator evaluates it. Through an adversarial training process, these networks continuously refine their capabilities. In BFSI, GANs can be applied in various innovative ways. For instance, they can be used to generate synthetic financial data for stress testing models or to create lifelike images for customer identification. GANs can also be harnessed for risk assessment by generating various scenarios to evaluate a financial institution’s resilience to market fluctuations.
- Natural Language Processing (NLP) and Contextual Understanding
Generative AI is not just about content generation; it’s about understanding and emulating human language. NLP plays a crucial role here. NLP models, such as transformer-based architectures like BERT and GPT, enable Generative AI to comprehend the intricacies of language, including context, sentiment, and semantics. This is invaluable in BFSI for tasks like automated customer support, financial report generation, and sentiment analysis for market insights.
Generative AI is not just an evolution; it’s a revolution. Its intricacies, powered by neural networks, GANs, and NLP, enable BFSI institutions to tap into uncharted territory, creating a future where the boundaries between human and AI capabilities blur, ultimately reshaping the landscape of the financial industry. Here are seven ways how.
Seven Pioneering Applications of Generative AI in the Finance Sector
Beyond the traditional applications that have been harnessed for some time, this cutting-edge technology is venturing into uncharted territories, offering unprecedented solutions that challenge the conventional wisdom of finance. From dynamic portfolio stress testing to AI-powered narrative generation, these applications epitomize the next frontier of financial innovation, promising a future where data-driven decisions and customer-centric services are taken to astonishing new heights. Here are the top seven that promise to make a difference:
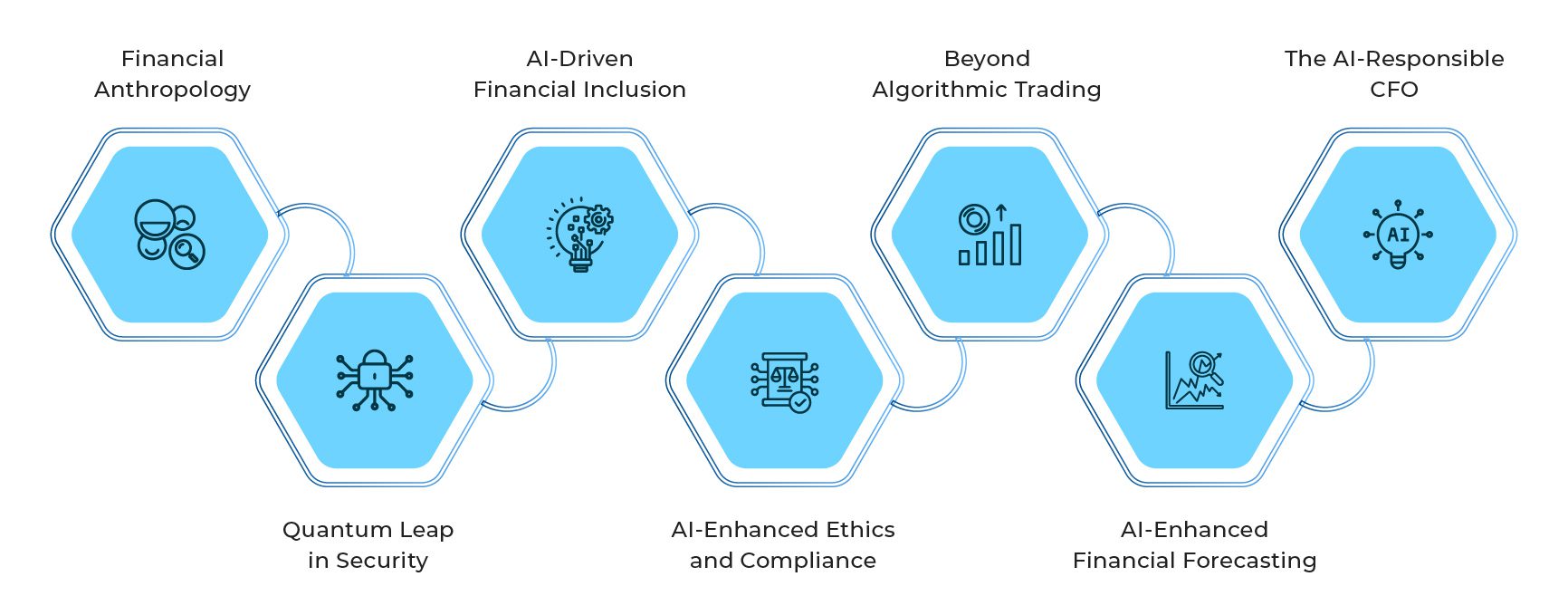
- Financial Anthropology
Understanding the Human Element: While AI in finance often conjures images of automated trading and algorithms, Generative AI is now diving deeper into the human side of finance. It is unraveling the intricate web of human behavior and emotions that influence financial decisions using advanced deep learning architectures, including Recurrent Neural Networks (RNNs) and Transformer models. By analyzing not just financial data but also social and psychological factors, AI is helping to predict market sentiment and anticipate the impact of societal events on financial markets. This newfound understanding is invaluable for investors and financial institutions to make more informed decisions. According to a study by Forrester Research, 78% of financial institutions have increased their investments in AI-driven sentiment analysis, highlighting its growing importance in understanding market sentiment.
- Quantum Leap in Security
Quantum-Safe Encryption: In an era of evolving cyber threats, Generative AI is spearheading a quantum leap in data security. While conventional encryption methods may become vulnerable to quantum computing, AI is at the forefront of developing quantum-resistant cryptographic algorithms. These techniques, underpinned by mathematical innovations like lattice-based cryptography, secure sensitive financial data against potential threats from quantum computing. According to a report by PwC, 58% of financial institutions are actively exploring quantum-safe encryption solutions.
- AI-Driven Financial Inclusion
Bridging the Financial Gap: Generative AI is redefining credit scoring with the aid of sophisticated machine learning algorithms, including Gradient Boosting and Neural Networks. By considering factors such as social media behavior, online activity, and even geospatial data, AI is enabling more accurate assessments of creditworthiness for individuals who were previously excluded from the formal financial system. This breakthrough is reshaping the landscape of lending and democratizing access to financial services. A study by the World Bank suggests that AI-driven alternative data sources have the potential to extend financial services to over 1.7 billion unbanked individuals.
- AI-Enhanced Ethics and Compliance
The Ethical Compliance Guardian: The financial industry’s Achilles’ heel has often been ethical breaches and non-compliance. It employs Natural Language Processing (NLP) models like BERT and GPT-3, combined with deep learning techniques, to scrutinize complex legal documents and financial communication. These models, trained on vast corpora of text data, have the capacity to discern subtle nuances in language, enabling them to identify potential compliance issues and ethical breaches before they become significant liabilities. According to a survey by Deloitte, 88% of financial executives consider AI-driven compliance monitoring crucial for their organizations.
- Beyond Algorithmic Trading:
AI as Financial Strategist: While algorithmic trading is well-known, Generative AI is shifting from mere execution to strategy formulation. Deep Reinforcement Learning (DRL) and Recurrent Neural Networks (RNNs) enable AI to create and manage complex trading strategies. The technical prowess of DRL allows AI to dynamically generate and optimize trading strategies by continuously monitoring real-time market conditions, news, and economic indicators. These strategies adapt dynamically to unfolding events, providing investors with a level of agility and responsiveness that was once inconceivable. According to a report by JPMorgan Chase, AI-driven trading strategies have outperformed traditional approaches by 30% in recent years.
- AI-Enhanced Financial Forecasting:
Anticipating Global Economic Trends: Generative AI is transcending traditional financial forecasting. It’s not just about predicting individual stock prices but about providing comprehensive insights into global economic trends. Gen AI employs advanced neural network architectures such as Convolutional Neural Networks (CNNs) and Gated Recurrent Units (GRUs) for financial forecasting. The combination of CNNs for feature extraction and GRUs for sequence modeling enables AI to reveal hidden correlations and causal relationships between economic events, geopolitical factors, and financial markets. A report by McKinsey & Company highlights that AI-enhanced financial forecasting has reduced forecasting errors by up to 25%.
- The AI-Responsible CFO:
Transforming Financial Leadership: Beyond data analysis, Generative AI is stepping into the realm of strategic financial leadership. This is made possible by a combination of advanced deep learning techniques, including Long Short-Term Memory (LSTM) networks that process real-time data, offering insights into risk management, resource allocation, and financial strategy optimization. It’s not just about automating financial tasks but about redefining the role of the CFO as a data-driven strategist. According to a survey by KPMG, 74% of CFOs consider AI to be a key enabler for strategic decision-making in their organizations.
Five Enterprises Transforming the Landscape with Gen AI
- Kavout: Kavout, a financial technology company, leverages Generative AI to enhance trading strategies and stock analysis. Their AI-powered platform, K Score, uses natural language processing and deep learning algorithms to process large volumes of financial news and data, generating predictive stock scores that aid traders and investors in making more informed decisions.
- JP Morgan Chase: One of the largest banks globally, JP Morgan Chase, employs Generative AI for various applications, including risk management, fraud detection, and trading strategies. The bank has developed AI-powered algorithms that continuously analyze market data and events, allowing for more precise trading decisions and efficient risk assessment.
- Goldman Sachs: Goldman Sachs has incorporated Generative AI into its asset management division. They use AI algorithms to process and analyze financial news, economic indicators, and market trends, generating real-time insights that aid asset allocation and investment decision-making.
- American Express: American Express utilizes Generative AI in its fraud detection and prevention efforts. The company employs machine learning algorithms that analyze transaction data and customer behavior, enabling real-time fraud detection and reducing false positives, thereby improving the customer experience.
- Citigroup: Citigroup leverages Generative AI for natural language processing to enhance customer service and streamline client inquiries. Using AI-powered chatbots and virtual assistants, Citigroup provides immediate, personalized responses to customer questions, ultimately improving customer satisfaction and reducing operational costs.
The Significance of Effective Data Management and Utilization with Generative AI
Efficient and intelligent data management and utilization are the lifeblood of Gen AI’s success in the dynamic realm of BFSI. Beyond the obvious advantages of data-driven decision-making, it’s the intricate tapestry of interconnected data that holds the keys to innovation. Gen AI thrives not just on structured financial data, but it’s the unconventional gems hidden within unstructured data sources that fuel its transformative potential. Here are seven steps to help enterprises lay the foundation for an efficient and intelligent data management ecosystem.
- Data Integration and Aggregation: Efficient data integration and aggregation are not mere technical processes; they are the keystones of Gen AI’s ability to grasp the holistic financial landscape. By drawing from structured databases, unstructured social media feeds, and real-time market data, Gen AI is armed with a comprehensive dataset that transcends silos. Implementation involves using ETL (Extract, Transform, Load) tools and platforms. For instance, Apache Nifi provides a scalable, open-source solution to ingest data from diverse sources, performing real-time transformations. Data lakes are established, often on Hadoop clusters or cloud-based solutions like AWS S3. Data is structured using schema-on-read techniques, ensuring flexibility in handling unstructured and semi-structured data. This empowers us to make well-informed, holistic decisions, resulting in superior risk assessments, more precise financial predictions, and a depth of market understanding that shapes BFSI into a dynamic and customer-centric industry.
- Data Quality and Cleansing: The intricate balance of data quality and cleansing is a symphony that elevates Gen AI’s capabilities. It can be realized through automated data quality checks and validation scripts that meticulously comb through data, ensuring that inaccuracies and inconsistencies are identified and rectified. Tools like Apache Spark and its DataFrame API allow data profiling and deduplication. Anomaly detection models, such as Isolation Forests or Autoencoders, can automatically identify inconsistencies and outliers in large datasets. Machine learning-based models can be developed and deployed using frameworks like Scikit-Learn or PyTorch. The benefits are manifold. Gen AI, fueled by clean data, generates more precise insights, effectively reducing errors and enhancing the quality of its outputs. This translates to improved risk assessment, more vigilant fraud detection, and exceptional customer service, cultivating a culture of trust and unparalleled customer satisfaction.
- Data Security and Privacy: The fortification of data security and privacy is a fortress around Gen AI’s operation in BFSI. It deploys encryption mechanisms like Advanced Encryption Standard (AES-256) for data at rest and Secure Sockets Layer/Transport Layer Security (SSL/TLS) for data in transit. Blockchain technology can be employed for securing transaction data. Access control is enforced through IAM systems, such as AWS Identity and Access Management (IAM) or Keycloak. AI-driven compliance monitoring is implemented through machine learning models that continuously analyze data for anomalies and compliance violations, with tools like Splunk or Elastic Stack for log analysis. With AI-driven compliance monitoring, Gen AI operates within the tightest legal bounds, ensuring data breaches are prevented and privacy regulations are scrupulously adhered to. The benefits of this technical vigilance are manifold, encompassing the protection of sensitive financial data, the preservation of an institution’s reputation, and the trust and satisfaction of its customers.
- Data Contextualization: Textual data is a treasure trove of insight, but only when the context is unlocked. Gen AI depends on advanced Natural Language Processing (NLP) models, fine-tuned on industry-specific data. Pre-trained models like BERT or GPT-3 are fine-tuned on data for tasks like sentiment analysis, entity recognition, and semantic analysis. Text data preprocessing uses Python libraries like spaCy for tokenization and sci-kit-learn for feature extraction. Contextual information, such as market events or customer interactions, is integrated with historical data to provide Gen AI-rich context. The result is more accurate sentiment analysis, deeper customer interactions, and a nuanced understanding of market trends. BFSI institutions respond agilely to market dynamics, offering tailored services and anticipating customer needs.
- Advanced Analytics and Machine Learning: The labyrinth of advanced analytics and machine learning forms the heart of Gen AI’s intelligence. Predictive modeling, implemented with deep learning frameworks like TensorFlow and PyTorch, empowers Gen AI to forecast market trends and make informed decisions. Machine learning pipelines are established with tools like Apache Airflow to ensure seamless model training and deployment. Anomaly detection algorithms like Isolation Forests and One-Class SVMs, trained on historical data, offer real-time vigilance against irregularities. Reinforcement learning models, woven with the technical marvels of frameworks like OpenAI’s Gym, allow Gen AI to adapt to evolving data and market conditions. Additionally, reinforcement learning models, often built using OpenAI’s Gym or TensorFlow, enable Gen AI to adapt to evolving data and market conditions, allowing it to make real-time decisions. The outcome is twofold: precise risk assessments, dynamic trading strategies, and real-time decision-making. This sets a stage where financial performance is optimized, and BFSI institutions gain a competitive edge in the market.
Innovative data management practices are pivotal in unleashing the full potential of Generative AI in the finance sector. As Generative AI continues to evolve and disrupt traditional financial practices, institutions prioritizing comprehensive and forward-thinking data management will be better positioned to thrive in the data-driven, customer-centric, and highly efficient future of finance.
The Data Dynamics Advantage
In the realm of data management for Generative AI in the finance sector, the burgeoning volumes of unstructured data present a formidable challenge. As financial institutions accumulate extensive data from diverse sources, the imperative of devising a highly effective strategy for the organization and management of this information cannot be overstated.
The selection of the most appropriate data management approach, whether it entails building an internal data team or collaborating with an external vendor, holds profound implications for operational efficiency and overall financial profitability. While initially appearing as a daunting undertaking, mastering the expansion of unstructured data positions financial organizations ahead of competitors grappling with disjointed and inefficient data management systems. It is precisely in this arena that Data Dynamics excels.
Data Dynamics emerges as a preeminent provider of enterprise data management solutions, extending a lifeline to financial institutions navigating the complexities of unstructured data through its Unified Unstructured Data Management Platform. This all-encompassing platform incorporates four pivotal modules: Data Analytics, Mobility, Security, and Compliance.
Proven effective in over 28 Fortune 100 organizations, the Data Dynamics Platform is fortified by a fusion of automation, Artificial Intelligence (AI), Machine Learning (ML), and blockchain technologies. It effortlessly scales to meet the demands of global enterprise workloads. With Data Dynamics as their partner, financial institutions can bid adieu to fragmented, point-based solutions and disparate data perspectives. Instead, they can wholeheartedly embrace a unified software platform that not only brings structure to unstructured data but also unlocks priceless data-driven insights, bolsters data security, guarantees compliance and governance, and spearheads initiatives related to cloud data management.
Ultimately, Data Dynamics envisions a future where financial institutions attain data democratization, allowing users of diverse technical backgrounds to instantaneously access, comprehend, and extract maximum insights from sprawling, unstructured data landscapes.
To delve deeper into how Data Dynamics can empower your enterprise in structuring unstructured data and optimizing costs, please explore our offerings at www.datadynamicsinc.com or reach out to us at solutions@datdyn.com or (713)-491-4298.