- A counterintuitive trend is emerging: cloud repatriation, where companies are moving some workloads back on-premises. This shift is driven by a complex interplay of factors, with cost optimization emerging as a primary catalyst.
- Outdated infrastructure and legacy systems are hindering enterprise cloud journey. To unlock the full potential of the cloud, organizations must shed these constraints and embrace digital transformation.
- Data analytics is the cornerstone of a successful cloud migration. By understanding data usage patterns, dependencies, and quality, enterprises can optimize their cloud infrastructure, reduce costs, and enhance performance.
- A significant hurdle to data-driven cloud migration is the prevalence of unstructured data, which accounts for approximately 80% of enterprise data. Extracting actionable insights from this vast, unorganized information requires sophisticated techniques and tools capable of analyzing diverse data sources.
The cloud computing landscape is evolving rapidly as enterprises accelerate their digital transformation initiatives. Gartner’s prediction of a 23.1% surge in global end-user spending on public cloud services to $525 billion by the end of 2024 underscores the industry’s vitality. Cloud platforms are becoming the bedrock for innovative applications, with AI and machine learning at the forefront.
However, a counterintuitive trend is emerging: cloud repatriation, where companies are moving some workloads back on-premises. This shift is driven by a complex interplay of factors, with cost optimization emerging as a primary catalyst. While cloud providers offer economies of scale, many enterprises grapple with unexpected expenses due to the consumption-based pricing models. A recent survey revealed that nearly half of organizations face unforeseen costs on public cloud platforms, prompting a critical reassessment of cloud strategies.
Performance challenges also contribute to the repatriation wave. Applications optimized for traditional infrastructure often encounter latency and bandwidth bottlenecks in public cloud settings. This is particularly evident in high-performance computing (HPC) domains, where low-latency and high throughput are paramount. To address these issues, companies like Dropbox have invested in custom-built data centers, demonstrating the potential benefits of tailored infrastructure.
Security and compliance remain formidable obstacles to cloud adoption. The stringent data protection regulations prevalent in various industries necessitate granular control over data handling. Public cloud environments, while offering robust security features, may not always meet the stringent requirements of sectors like finance and healthcare. Consequently, institutions such as Bank of America are opting for private cloud solutions to safeguard sensitive information and ensure compliance with regulations like GDPR and CCPA.
At the heart of this challenge lies a critical oversight: the underestimation of data analytics in cloud adoption. This shortcut often leads to unexpected consequences. At the heart of this challenge lies a critical oversight: the underestimation of data analytics in cloud adoption. Many enterprises, in their haste to reap the benefits of the cloud, opt for a “lift and shift” approach, simply migrating their applications and data without a comprehensive analysis. This shortcut often leads to unexpected consequences.
The Pitfalls of Lift-and-Shift Cloud Migration
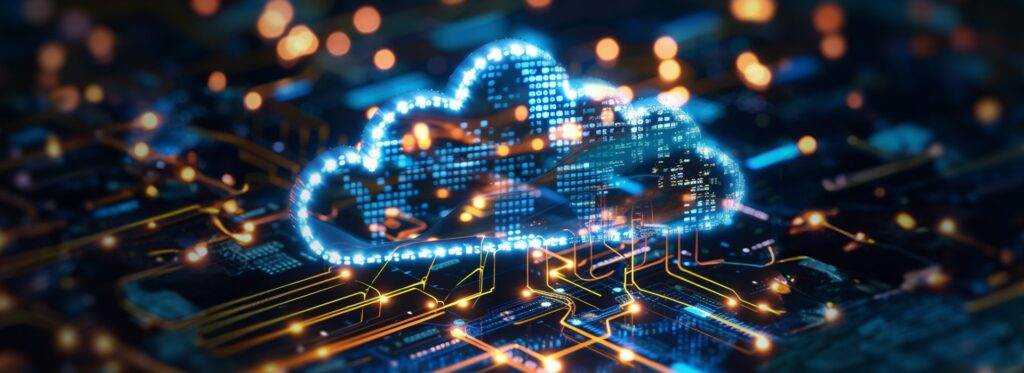
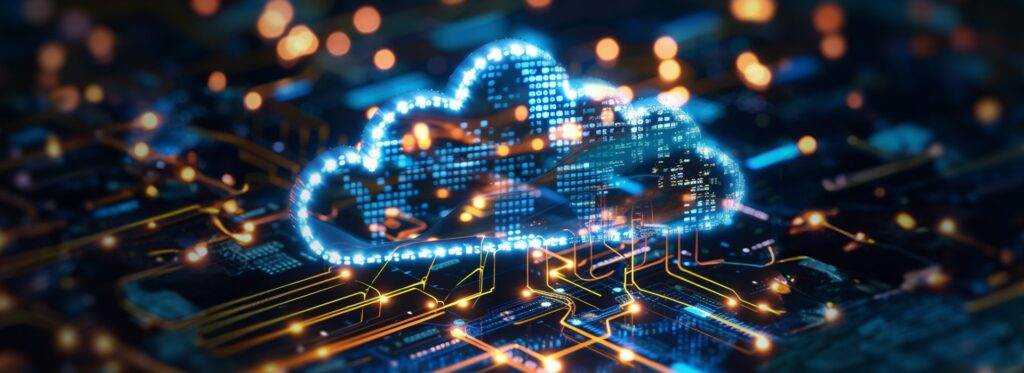
The lift-and-shift approach, which involves migrating applications and data to the cloud with minimal adjustments, often leads to several technical and operational challenges. This strategy overlooks the inherent differences between on-premises and cloud environments, leading to performance inefficiencies and cost escalations. Applications designed for traditional infrastructure may fail to utilize cloud-native features, such as auto-scaling and serverless architectures, resulting in underutilized or over-provisioned resources.
Furthermore, lift-and-shift migrations typically neglect the need for data transformation and integration. This oversight can create fragmented data ecosystems, complicating data governance and compliance. Inadequate optimization and integration can lead to data management issues, increased security vulnerabilities, and challenges in maintaining regulatory compliance.
In the era of AI and advanced analytics, the lift-and-shift approach is increasingly inadequate. Modern AI and machine learning technologies require specific architectural optimizations and integrations that are not addressed by a simple lift-and-shift strategy. Cloud platforms offer a range of AI services, such as natural language processing (NLP), image recognition, and predictive analytics, which necessitate application modifications to fully leverage these capabilities.
For instance, integrating with platforms offering AI services requires applications to be re-architected to interact with these specialized tools. Without such modifications, organizations may struggle to exploit the full potential of AI technologies, limiting innovation and competitive advantage. Additionally, data privacy and compliance requirements, such as GDPR and CCPA, demand robust data handling and protection measures that are often overlooked in a lift-and-shift approach.
Data Analytics as the Foundation for Cloud Migration
Data analytics is the cornerstone of a successful cloud migration. By understanding data usage patterns, dependencies, and quality, enterprises can optimize their cloud infrastructure, reduce costs, and enhance performance. Moreover, data analytics is essential for ensuring data privacy and security. By identifying sensitive data and implementing appropriate protection measures, organizations can mitigate the risk of data breaches and comply with regulations like GDPR and CCPA. Let’s find out more.
- Data Inventory and Classification: A detailed data inventory and classification process is critical. This involves cataloging all data assets and categorizing them based on sensitivity, value, and usage patterns. Advanced techniques in data discovery and metadata management can automate the scanning of data sources to create a comprehensive repository. This classification is crucial for prioritizing data migration efforts, ensuring that sensitive and high-value data is protected and compliant throughout the migration process.
- Dependency Mapping and Application Discovery: Understanding application dependencies and data flows is essential for effective migration. Dependency mapping provides a detailed view of how applications and data interact, helping to identify potential risks and interdependencies that could impact the migration. This visibility aids in planning migrations in a coordinated manner, ensuring that data and applications are moved with minimal disruption and maintaining application performance and functionality post-migration.
- Data Quality Assessment and Transformation: Assessing data quality is vital to ensure that data being migrated is accurate, complete, and consistent. Data profiling techniques analyze data quality metrics, identifying issues such as duplicates, inconsistencies, and inaccuracies. This assessment supports data cleansing and transformation efforts, preparing data for migration by ensuring it meets quality standards and is aligned with cloud requirements. Transformation processes may include normalization, deduplication, and enrichment to optimize data for cloud environments.
- Performance Benchmarking and Optimization: Benchmarking current system performance is crucial for identifying potential bottlenecks and areas for improvement. Performance monitoring and analysis help in understanding existing application performance and resource utilization. This information is used to optimize applications and infrastructure for the cloud, ensuring they leverage cloud-native features effectively. Performance optimization may involve re-architecting applications to utilize cloud services, such as integrating with cloud-native databases or adopting microservices architectures.
- Cost Analysis and Forecasting: Effective cloud cost management involves analyzing current usage patterns and forecasting future costs based on different cloud configurations. This analysis helps in making informed decisions about resource allocation and identifying opportunities for cost savings. Understanding the cost implications of various cloud configurations and pricing models ensures that resources are used efficiently and prevents unexpected expenses.
- Compliance and Security Integration: Ensuring compliance with data protection regulations and implementing robust security measures are essential for a successful cloud migration. Data analytics plays a key role in assessing compliance requirements and integrating security controls. This includes implementing encryption, access controls, and continuous monitoring to protect data and ensure it meets regulatory standards throughout its lifecycle.
While these steps outline a clear path to data-driven cloud migration, the reality is more complex. A significant challenge lies in the vast amount of unstructured data that constitutes about 80% of enterprise data. This unstructured data, often likened to a black hole of information, poses significant hurdles in data analysis and migration. Transforming this unstructured data into actionable insights requires advanced techniques and tools that can extract meaningful information from diverse sources. Overcoming this challenge is crucial for unlocking the full potential of data-driven cloud migration.
Five Unique Points for Data-Driven Cloud Adoption
- Dynamic Data Classification Using AI Algorithms
Employing advanced machine learning algorithms for real-time data classification can significantly enhance data management. Techniques like clustering and anomaly detection automate data categorization based on usage patterns and sensitivity. This not only improves data security by prioritizing critical data but also optimizes storage and retrieval. For instance, sensitive data can be encrypted and stored in secure environments, while less critical data can be stored in more cost-effective tiers.
- Predictive Analytics for Hybrid Cloud Optimization
Predictive analytics can revolutionize hybrid cloud management by forecasting performance and cost implications of different configurations. By analyzing historical data and usage patterns, organizations can proactively adjust workload distribution between public and private clouds. This optimization leads to cost savings, improved performance, and enhanced resilience. For example, predicting peak usage times can allow for automatic scaling of resources in the public cloud, avoiding costly overprovisioning.
- Real-Time Data Governance with Automated Policy Enforcement
Implementing real-time data governance with automated policy enforcement is crucial for maintaining data integrity and compliance. Continuous monitoring of data access and usage, coupled with automated policy enforcement, ensures adherence to regulations like GDPR and CCPA. This proactive approach helps prevent data breaches, reduces the risk of fines, and builds trust with customers. For instance, automated detection of anomalous data access patterns can trigger alerts and investigations, preventing potential security incidents.
- AI-Enhanced Data Insights for Strategic Decision-Making
Leveraging AI-driven analytics platforms can unlock hidden value within vast datasets. By identifying trends, patterns, and correlations, organizations can make data-driven decisions that drive innovation and competitive advantage. For example, AI can analyze customer behavior patterns to identify new market opportunities or optimize marketing campaigns. Additionally, predictive analytics can forecast future trends, enabling businesses to proactively adapt to changing market conditions.
- Continuous Optimization Through Automated Resource Management
Adopting automated resource management strategies is essential for maximizing cloud efficiency and cost-effectiveness. Real-time analytics provide insights into resource utilization and performance, enabling dynamic adjustments. Automated scaling and load balancing optimize resource allocation, ensuring optimal performance while minimizing costs. For example, by automatically scaling resources based on workload fluctuations, organizations can avoid overprovisioning and reduce cloud expenses.
As cloud adoption accelerates, a strategic, data-driven approach to migration becomes increasingly critical. Moving beyond the lift-and-shift strategy and embracing data analytics as the foundation for cloud migration is essential for optimizing cloud strategies and achieving long-term success. Data Dynamics provides the insights necessary to navigate the complexities of cloud adoption, ensuring that businesses can harness advanced technologies while maintaining control over their data.
At the core of this transformation lies our Unified Data Management Software, tailored to address three pivotal aspects crucial for the success of cloud migration. Firstly, leveraging advanced data visualization, the software extracts actionable insights, providing decision-makers with a dynamic view of their data landscape. This not only enhances optimization but also facilitates informed decision-making, steering organizations toward more efficient operations and resource utilization throughout the migration journey. Secondly, the software places a strong emphasis on elevating data utilization, availability, and accessibility. By simplifying data access and utilization, not only breaks down silos but also ensures that information is readily available to support the migration process. This not only enhances productivity but also fosters collaboration across different teams, creating a more agile and responsive organizational environment during the critical transition to the cloud. Moreover, the software goes beyond traditional measures by proactively addressing the challenges of data sprawl and cyber resilience. Robust data governance and security measures are seamlessly integrated to reduce risks associated with unauthorized access, data breaches, and cyber threats. This comprehensive approach not only safeguards sensitive and consumer data but also ensures regulatory compliance, providing organizations with a secure foundation as they embark on their cloud migration journey. In addition to these core aspects, the software introduces intelligent data placement to streamline resource allocation in the cloud environment. These advanced capabilities empower organizations to navigate the evolving landscape of digital transformation with confidence and agility.
To learn more about how Data Dynamics can empower your organization to navigate the complexities of cloud migration with confidence, visit www.datadynamicsinc.com or contact us at solutions@datdyn.com or (713)-491-4298.