In the rush to integrate artificial intelligence across every function—from cyber threat detection to fraud mitigation—CISOs are finding themselves on the frontlines of transformation. AI isn’t just a capability; it’s a competitive differentiator. But here’s the uncomfortable truth: while enterprises continue to pour billions into AI models and infrastructure, many are ignoring the critical prerequisite for success—data readiness.
According to Deloitte, up to 80% of the time and resources in AI projects go toward data preparation, yet most of that effort is reactive, fragmented, and often insufficient. The result? AI models trained on incomplete, misclassified, or non-compliant data that produce unpredictable or even dangerous outcomes. As threats become more sophisticated and compliance becomes more unforgiving, the message for CISOs is clear: AI strategy is data strategy—and it starts with readiness.
What CISOs Need to Know About Data Readiness
Data readiness goes far beyond hygiene. It’s about having complete control over the quality, context, classification, accessibility, and ethical governance of data. For a CISO, that translates directly into risk mitigation, policy enforcement, and operational trust.
Data readiness includes:
- End-to-end visibility: Knowing what data exists, where it lives, and who has access.
- Risk-aware classification: Identifying sensitive data, especially unstructured, for appropriate protections.
- Policy-based governance: Automating controls and access based on security posture.
- Ethical and compliant usage: Ensuring that data feeding AI respects privacy laws and organizational integrity.
AI is a multiplier, but it amplifies both signal and noise. And the noise, when unchecked, leads to breaches, hallucinations, or biased decisions.
The Rising Risk of Unstructured Data
Here’s where it gets more urgent: the majority of enterprise data is unstructured. Emails, images, PDFs, source code, and collaboration docs—this data is often scattered across systems, poorly governed, and rich with potential exposure points.
Yet it’s exactly this kind of data that modern AI models crave. LLMs and SLMs extract insights from unstructured information to make decisions, recommend actions, and automate workflows. Without proper readiness protocols, CISOs risk letting sensitive or toxic data into the model training process.
The consequences?
- Models trained on old or corrupted documents
- Shadow AI deployments in business units
- Data leakage through overly permissive access
- Bias stemming from non-contextualized inputs
AI Failures Often Begin with Data Failures
Consider this: a model fails to identify a security breach because log files weren’t properly parsed. Another generates biased hiring recommendations because HR documents were inconsistently tagged. A predictive system overestimates customer churn due to outdated financial data.
For CISOs, that means focusing less on adjusting AI models and more on strengthening the data supply chain that fuels them.
The CISO’s Playbook for Data-Ready AI
As organizations scale AI investments, CISOs must move beyond traditional notions of perimeter defense and into the realm of data governance leadership. The role is no longer confined to securing infrastructure—it now includes securing what powers AI: the data itself.Here’s a comprehensive playbook for CISOs to enable secure, compliant, and trustworthy AI through effective data readiness:
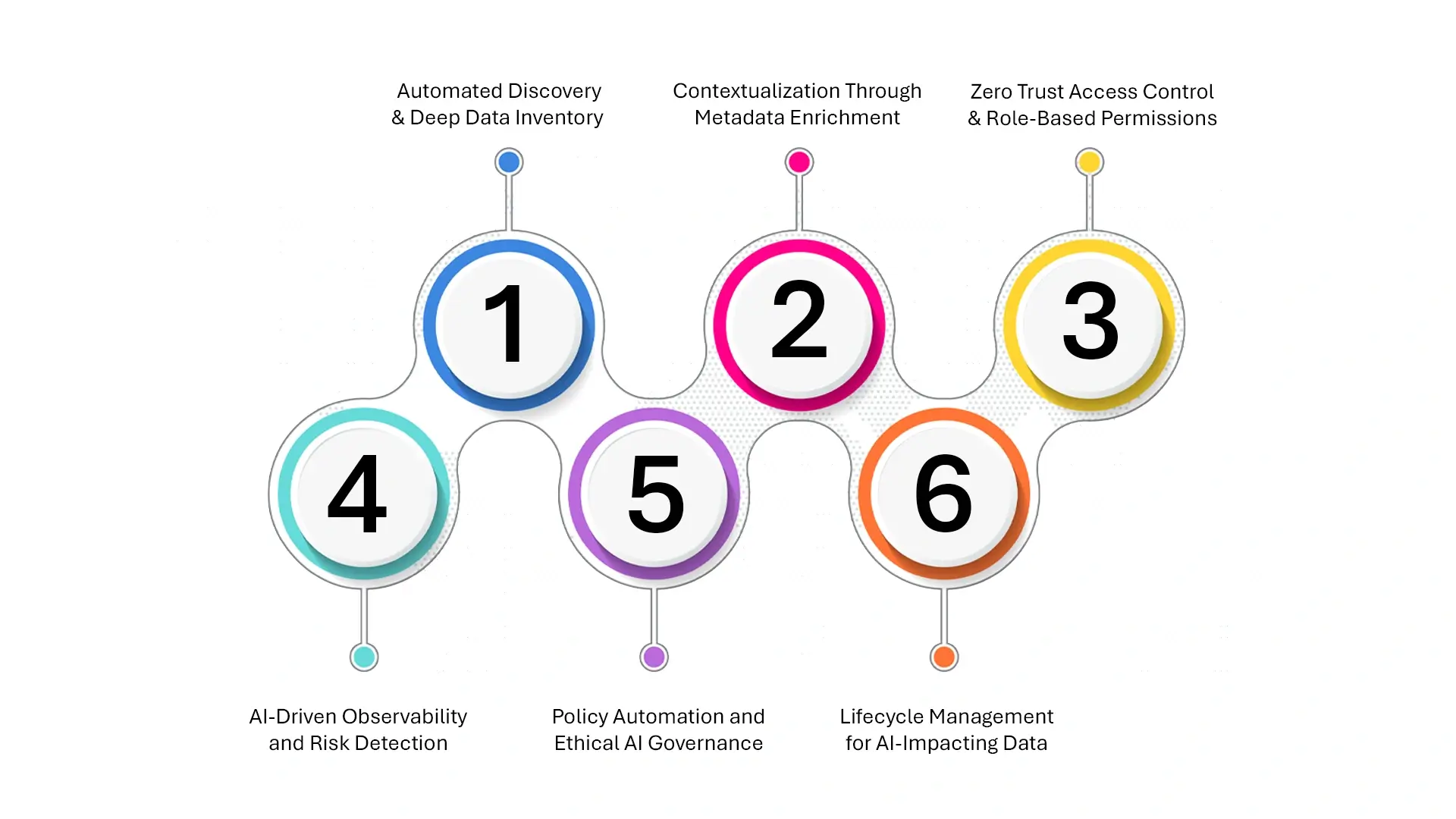
1. Automated Discovery & Deep Data Inventory
The first step to managing risk is knowing what you’re dealing with. That means establishing real-time visibility into enterprise-wide data, including structured databases, file repositories, cloud drives, messaging platforms, and collaborative tools.
CISOs should:
- Deploy AI-powered discovery tools that scan across hybrid environments
- Identify sensitive data types, such as PII, PHI, financial records, or intellectual property
- Flag data residing in high-risk zones, such as unsecured shares or shadow IT repositories
Why it matters: Without a clear inventory, unclassified and unmanaged data can easily be ingested into AI models—introducing legal, ethical, and reputational risks.
2. Contextualization Through Metadata Enrichment
Discovery alone isn’t enough. Data must be contextualized to understand its relationships, usage, and relevance. Metadata enrichment tags files with key attributes—ownership, origin, sensitivity, retention needs, and business purpose.
CISOs should implement:
- Automated metadata tagging pipelines
- Integration with data catalogs and knowledge graphs
- Policy-based labeling for security and lifecycle management
Why it matters: Context-aware data ensures that AI models are trained on relevant, high-fidelity inputs—reducing errors, bias, and compliance gaps.
3. Zero Trust Access Control and Role-Based Permissions
Most access management frameworks focus on structured systems. But unstructured data often slips through the cracks, left wide open or poorly governed. That’s a breach waiting to happen.
CISOs must:
- Extend Zero Trust architecture to files, emails, and collaboration content
- Enforce Role-Based Access Control (RBAC) down to the file level
- Automate re-permissioning based on user roles, locations, and usage patterns
Why it matters: Misaligned access permissions can lead to internal threats or data leakage into AI pipelines, especially in federated learning environments where data moves rapidly between systems.
4. AI-Driven Observability and Risk Detection
Static controls are no longer sufficient. As AI models evolve and data flows become dynamic, security must become proactive and intelligent. Observability tools powered by machine learning provide real-time insights into anomalies, policy violations, and suspicious behaviors.
CISOs should:
- Monitor data usage patterns across all environments
- Detect drift in datasets used by AI pipelines
- Analyze file lineage and access trails for anomaly detection
Why it matters: AI-enabled observability allows CISOs to surface threats or exposure points before they compromise the AI lifecycle or trigger regulatory infractions.
5. Policy Automation and Ethical AI Governance
AI readiness isn’t just a technical function—it’s a governance mandate. Regulatory requirements like the EU AI Act and India’s DPDP Act are beginning to formalize what CISOs have long known: organizations must ensure ethical, explainable, and auditable AI.
CISOs should:
- Establish automated policy engines for data movement, access, and retention
- Maintain logs and evidence trails for model training datasets
- Enforce data minimization, purpose limitation, and audit readiness
Why it matters: Without clear policy enforcement, enterprises risk using non-compliant or unauthorized data in training AI, potentially triggering legal and financial consequences.
6. Lifecycle Management for AI-Impacting Data
Data used for AI isn’t static—it evolves, gets outdated, or becomes irrelevant. CISOs must implement lifecycle strategies that continuously assess data quality, relevance, and risk.
This includes:
- Automated archiving or deletion of obsolete datasets
- Regular validation of training datasets against current policies
- Alerting systems for expired or duplicated content
Why it matters: AI models trained on stale or non-contextual data are not just inefficient—they’re dangerous. They can produce flawed outputs, propagate bias, or expose the enterprise to unnecessary scrutiny.
Real ROI: From Risk Mitigation to Faster Innovation
According to Deloitte, poorly prepared data increases operational costs, delays AI timelines, and introduces regulatory vulnerabilities. By contrast, data readiness:
- Accelerates time-to-insight by enabling immediate, secure access to usable data
- Reduces costs tied to re-training models and post-deployment cleanups
- Strengthens governance and reduces the attack surface through proactive classification
- Improves model performance by feeding systems with high-quality, contextualized input
Put simply: the better your data, the smarter—and safer—your AI becomes.
Why CISOs Must Act Now
With global AI regulations like the EU AI Act and India’s DPDP Act mandating risk controls and data traceability, the window for reactive strategies is closing fast. Cybersecurity threats are escalating, and AI models are being used not just to analyze but to act. The stakes for secure and compliant AI have never been higher. Scalable data preparation frameworks are not only possible—they’re critical to success.
CISOs have a unique opportunity to lead this evolution, not as gatekeepers, but as enablers of trustworthy AI.
Enter Zubin: AI-Ready Data Management for Security Leaders
At Data Dynamics, we recognize that secure AI starts with secure data. That’s why we developed Zubin, an AI-powered, self-service data management software.
Zubin gives you:
- Enterprise-wide visibility across unstructured, semi-structured, and structured data
- AI-driven discovery and classification to surface dark data and reduce risk
- Policy-based governance and Zero Trust enforcement through automated access control
- Continuous observability and auditability for real-time compliance and ethical usage
- Automated metadata enrichment to support context-aware AI training and decision-making
With Zubin, CISOs can ensure their AI initiatives are built on a secure, compliant, and future-ready data foundation. In an AI-first world, the biggest security risk isn’t the model—it’s the data feeding it. For CISOs, that shifts the role from perimeter protector to strategic enabler of responsible innovation.
By prioritizing data readiness, you don’t just protect the enterprise—you future-proof it. Zubin is here to help you lead with confidence, turning fragmented, risky data ecosystems into governed, AI-ready engines of transformation.
Because in 2025, smart security is data-first security, and it starts with readiness.