What is Data Lifecycle Management?
This process implies the entire cycle of data handling from its very birth to archiving, from usage till erasure. A policy or best practice in any organization governs the whole management process with suitable practices so as to govern it, maintain, and safeguard it throughout the whole stage, along with optimizing the available storage and strictly adhering to regulatory compliance requirements. Thus, DLM optimizes organizations for effective data management, and reduced risk, and that data should stay accessible, correct, and secured during its period of usage.
Stages of Data Lifecycle Management (DLM)
To implement data lifecycle management properly, organizations should follow a well-structured process that ensures proper handling of data at every step. From the time data is created or obtained, it has to be classified, stored safely, and then made available for business operations to meet governance and compliance requirements. As data is processed and analyzed, security measures must be in place to protect against breaches and unauthorized access. Over time, inactive data should be archived efficiently to reduce storage costs, while regulatory policies dictate retention periods. Finally, when data reaches the end of its lifecycle, it must be securely deleted to prevent exposure and ensure compliance with legal frameworks.
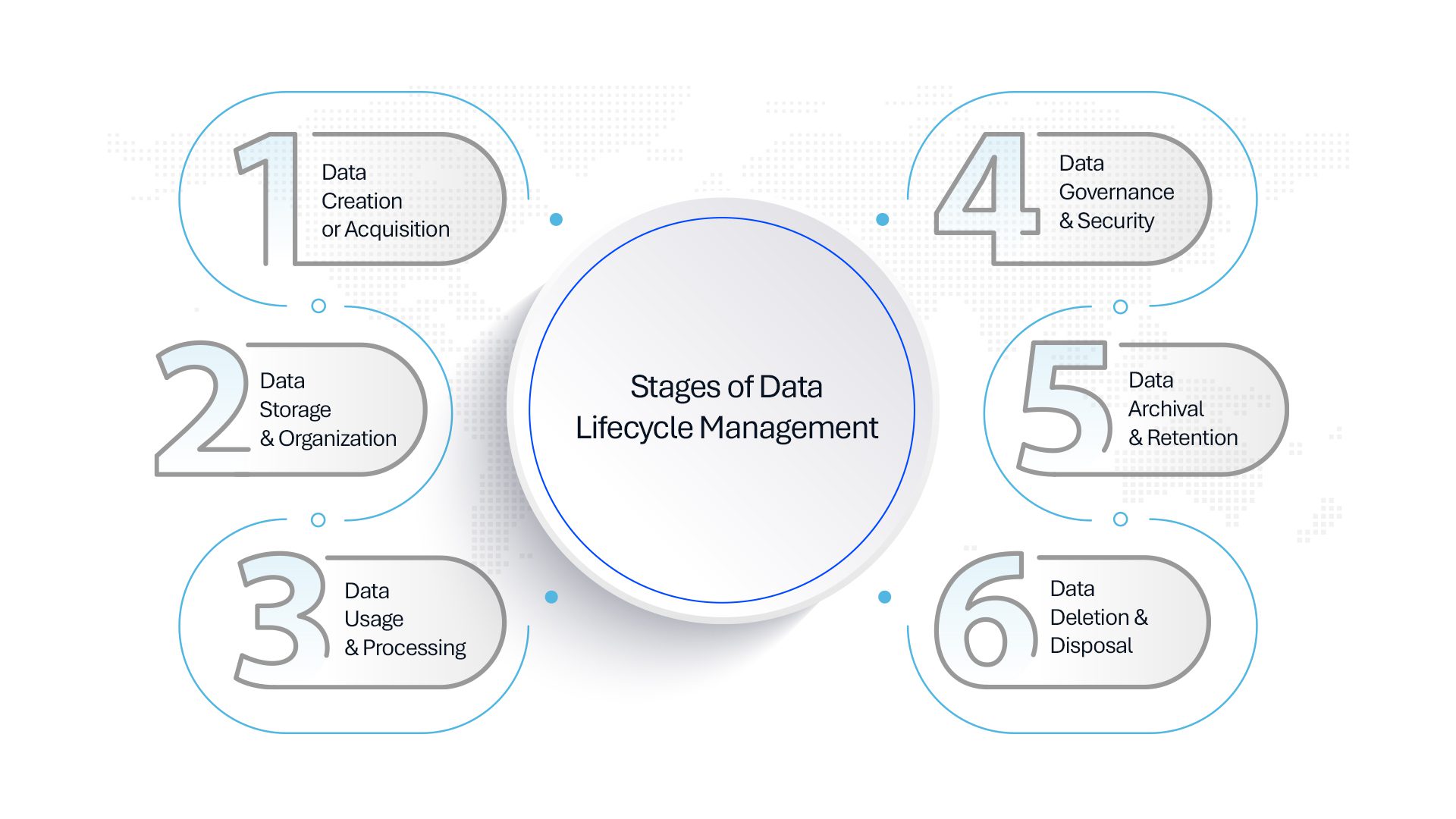
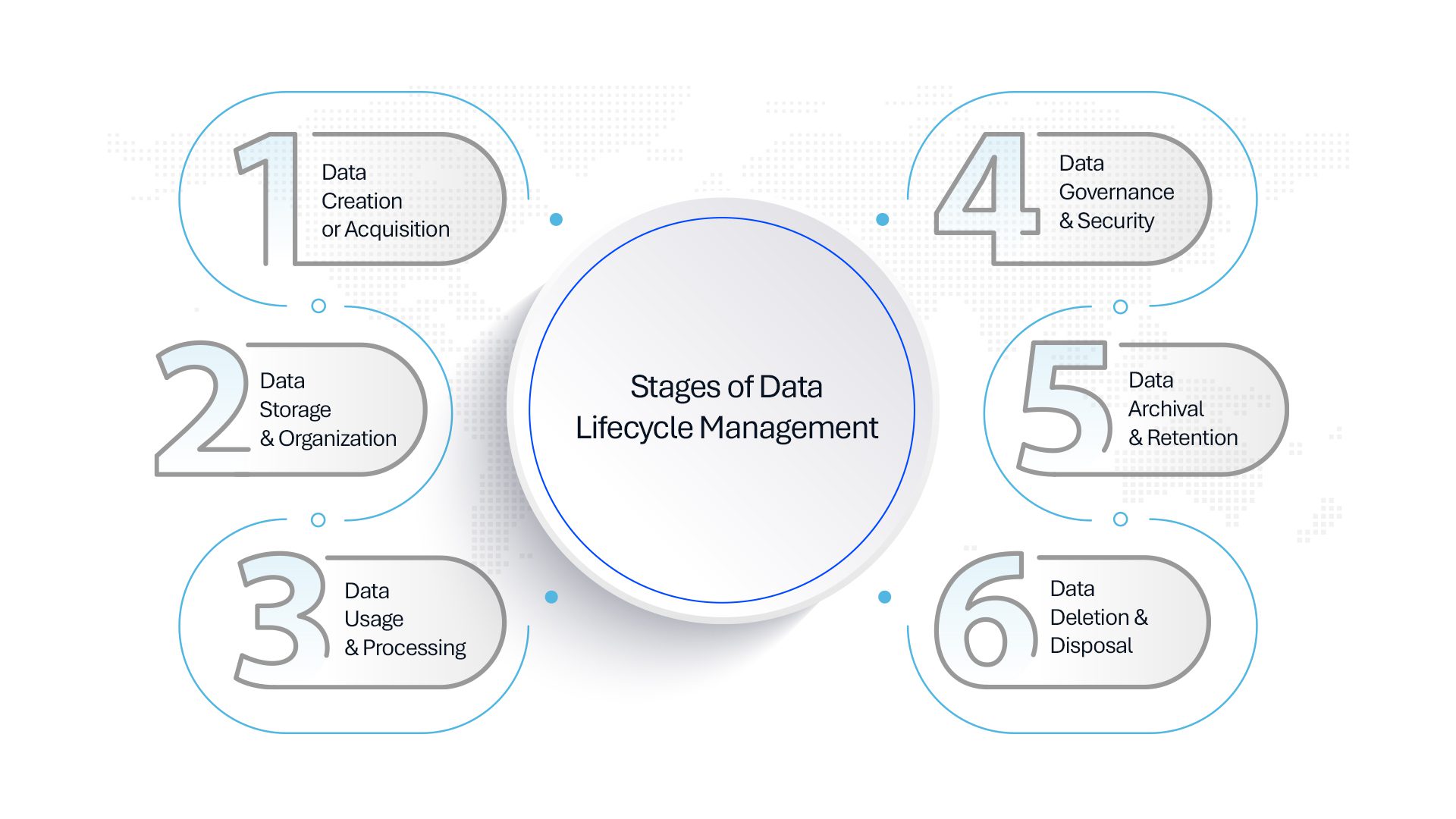
1. Data Creation or Acquisition
Data is created through enterprise applications, IoT devices, transactions, emails, and other external integrations. At this point, it is important to classify unstructured data, like emails, PDFs, multimedia. Metadata tagging helps define ownership, sensitivity, and business relevance, ensuring governance and compliance right from the beginning. Clear roles of data ownership with well-defined privacy policies are strong regulatory alignments. Initial validation processes detect duplicates, incomplete records, and inconsistencies, thereby improving data quality before it enters operational workflows.
2. Data Storage & Organization
Data must be stored efficiently in structured databases, data lakes, cloud repositories, or on-prem servers once it is created. Organizations use tiered storage models to distinguish between frequently used “hot data” and archival “cold data” to optimize performance and costs. Improved discoverability of data through metadata indexing and cataloging, while only authorized users gain access to sensitive data through the use of RBAC and ABAC. Encrypting information in rest and transit with AES-256, TLS, tokenization, and masking are some critical security measures in place. Alignment of storage locations with compliance mandates such as GDPR, HIPAA, and CCPA must ensure that data sovereignty laws are in place.
3. Data Usage & Processing
Data is busily being consumed by business intelligence, AI/ML analytics, and operational workflows. ETL (Extract, Transform, Load) as well as ELT pipelines enable frictionless data movement, while AI-driven analytics help unlock deeper insights from structured and unstructured datasets. Observability frameworks monitor real-time anomalies, unauthorized access, and performance bottlenecks at optimum data integrity. Self-service data access empowers teams with federated governance models, reducing IT dependency while maintaining security and control. Automated compliance monitoring ensures that data processing is compliant with regulatory frameworks and internal governance policies.
4. Data Governance & Security
Automated risk-scoring models categorize high-risk data, so organizations can ensure that the necessary security measures are in place accordingly. Data sovereignty laws dictate restrictions on geographical storage, ensuring that organizations comply with jurisdictional mandates such as GDPR and China’s CSL. Security frameworks like Zero Trust Security, multi-factor authentication (MFA), and conditional access policies prevent unauthorized access. AI-driven Data Security Posture Management (DSPM) and Data Loss Prevention (DLP) solutions continuously monitor for threats, reducing the risks of breaches. Real-time audit logs and compliance dashboards ensure transparent governance and accountability.
5. Data Archival & Retention
Data which isn’t accessed for a long time goes into long-term storage based on a set retention policy defined by businesses or regulatory requirements. Inactive data in this regard is stored cheaply in solutions like AWS Glacier or Azure Archive. AI-powered search tools make retrieval easier when search is required to ascertain the integrity of legal and compliance audits, supporting eDiscovery and forensic investigations. Organizations must be compliant with regulations like SOX, GDPR, and HIPAA, ensuring historical records are kept safely for the required retention period but are readily available when needed. Automated governance workflows simplify archival and retention compliance.
6. Data Deletion & Disposal
Once data surpasses its retention period, it must be securely deleted to prevent unauthorized access or regulatory violations. Automated data purging schedules notify stakeholders before deletion, ensuring compliance with internal and external requirements. Secure deletion techniques like cryptographic erasure, degaussing, and physical destruction permanently remove data from storage devices. Compliance frameworks like GDPR’s “Right to be Forgotten” require organizations to ensure the complete removal of all instances, including backups and logs. Final validation reports and certificates of destruction provide audit-proof evidence of compliance, ensuring organizations maintain transparency and accountability in their data lifecycle management.
Implementing a robust Data Lifecycle Management (DLM) strategy is essential for organizations to maintain data integrity, security, and compliance while optimizing storage and operational efficiency. By proactively managing data from creation to deletion, businesses can reduce risks, enhance decision-making, and ensure seamless governance across their data ecosystem. In an era of exponential data growth, a well-executed DLM approach is not just a necessity—it’s a competitive advantage.
Industry Insights and Trends
- The integration of Artificial Intelligence (AI) into DLM processes is on the rise, enabling automated data classification, improved analytics, and enhanced decision-making capabilities.
- According to IDC, 96% of organizations with mostly (or completely) siloed unstructured data don’t know what information lies inside their content.
- 29% of those surveyed by Accenture have “high trust” in their organization’s data, which is important to deriving value from it. By eliminating redundant, inaccurate, or obsolete information, DLM ensures your data is reliable, so you’re able to unlock actionable insights and gain a competitive advantage.
Getting Started with Data Dynamics:
Read the latest blog: Data Fabric and AI: The Blueprint for Dominating Unstructured Data in 2025
Learn about our Unstructured Data Management Software – Zubin
Schedule a demo with our team