In an era where artificial intelligence (AI) powers critical decision-making, data privacy and security have become paramount. Yet, as organizations amass unprecedented volumes of data to feed these intelligent systems, safeguarding information—especially unstructured data—becomes more complex. From personal identifiers embedded within documents to sensitive customer details, unstructured data represents both a high-value asset and a vulnerability.
To address these vulnerabilities, organizations must implement advanced privacy and security measures specifically tailored to the unique demands of AI systems. Traditional data protection methods fall short in an AI-driven environment, where unstructured data flows freely across platforms, often without clear boundaries. Implementing these advanced measures requires a clear understanding and control over the unstructured data that fuels AI systems. Let’s take a closer look.
Advanced Data Privacy and Security in the AI Era: Imperatives
- AI-Specific Privacy Concerns with Data Aggregation: AI requires aggregating data from multiple sources, often involving unstructured data that lacks standardized formats or metadata. This aggregation amplifies the risk of unintentional data exposure as data flows across systems without proper labeling or classification. Advanced data governance tools now employ AI-driven data classification that categorizes unstructured data in real time, tagging sensitive elements to ensure they’re handled according to privacy and compliance protocols.
- Differential Privacy for Protecting Sensitive Data in AI Models: As organizations leverage AI models, ensuring data privacy without sacrificing data utility is paramount. Techniques like differential privacy are being integrated directly into data pipelines, allowing models to learn from patterns in sensitive data without directly accessing identifiable information. This approach safeguards personal data in training datasets, ensuring that privacy is maintained even when data is extensively analyzed for AI purposes.
- Federated Learning for Enhanced Security and Privacy in Data Processing: Federated learning allows AI models to be trained across decentralized devices or servers holding local data samples without centralizing the raw data. By distributing the learning process, federated learning minimizes the need for data transfers and protects unstructured data at its source, reducing the risk of data leaks and exposure during transit.
- Automated Anonymization in Unstructured Data Workflows: Given that unstructured data often contains personal identifiers scattered across various formats, automated anonymization processes have become essential. These systems detect and scrub identifiers such as names, addresses, and social security numbers across document types, enabling compliance with data privacy standards while preserving the data’s analytical value.
- Adaptive Access Controls with Contextual Awareness: In modern AI-driven environments, security policies for unstructured data must adapt based on real-time context—such as user location, behavior, or data sensitivity. Adaptive access controls powered by AI monitor these contextual elements and dynamically adjust permissions. For example, if sensitive customer data is accessed from an unknown IP, the system can prompt multi-factor authentication or restrict access entirely, providing an intelligent layer of protection.
As organizations implement advanced measures to address AI-driven privacy and security challenges, it becomes evident that the effectiveness of these solutions hinges on a foundational element: unstructured data management. While AI techniques like differential privacy, federated learning, and adaptive access controls address the immediate need for safeguarding data, their success depends on an organization’s ability to identify, categorize, and control the unstructured data fueling these systems. Without a solid framework for managing unstructured data, even the most advanced tools can falter, leaving critical gaps in protection and compliance.
Transforming Chaos into Control: The Importance of Unstructured Data Management
Managing unstructured data begins with visibility—understanding what you have, where it resides, and how it’s classified. The challenge lies in the sheer complexity of this data: it’s scattered, unpredictable, and often hidden in formats that don’t lend themselves to easy categorization. To transform this chaos into control, organizations must harness tools that extract meaning from the unknown, uncovering the insights buried within.
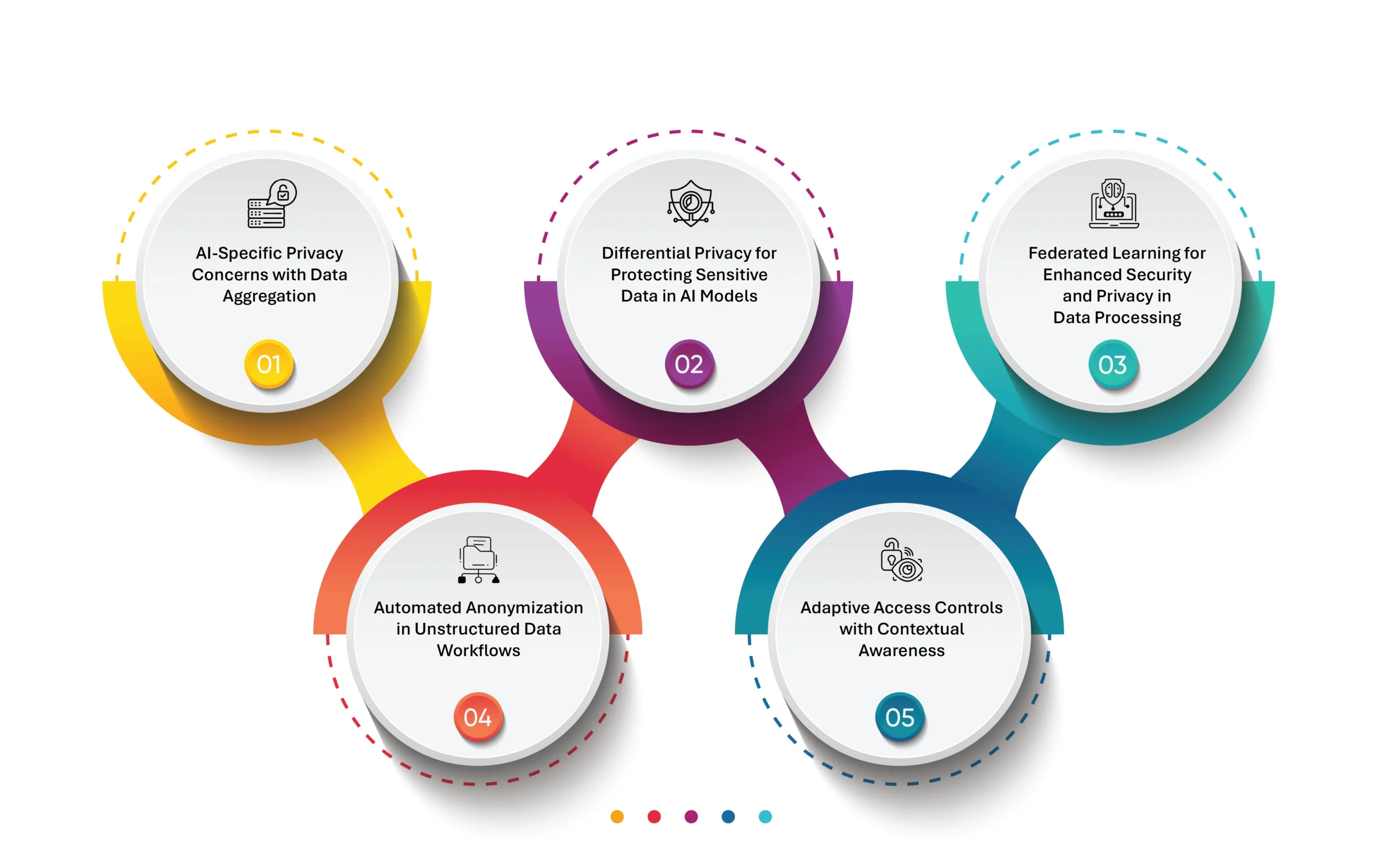
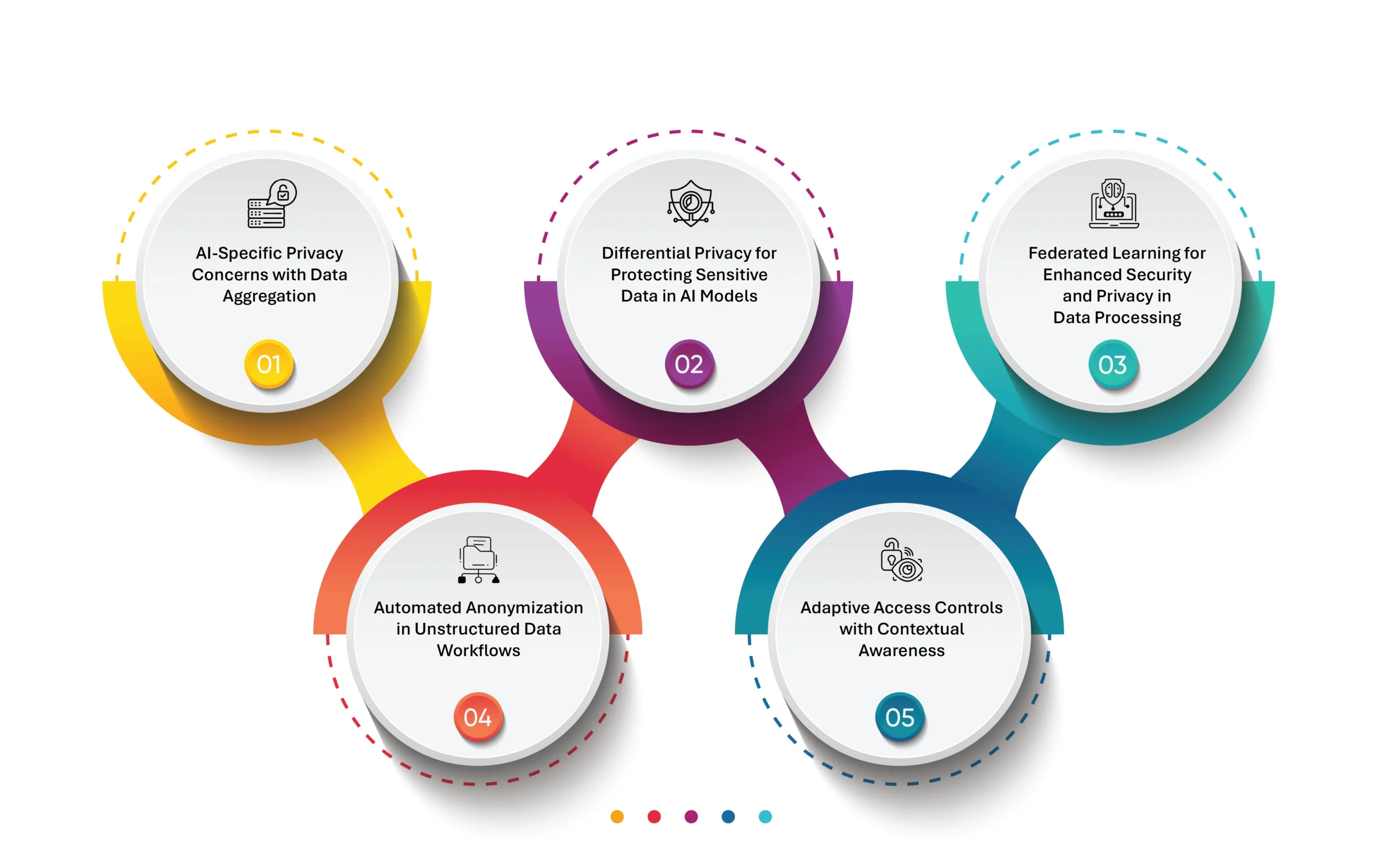
Metadata Extraction: The Compass for Navigating Your Data Ocean
Unstructured data is often a maze of unknowns, with crucial details buried deep within. It doesn’t self-identify as “important” or “sensitive,” leaving businesses with the impossible task of finding needles in haystacks. That’s where metadata extraction powered by AI becomes a game-changer. Metadata captures the DNA of a file—details like its creator, date of origin, and content highlights. AI tools take this further by parsing through mountains of documents and pulling out actionable insights such as names, financial figures, or locations. Picture an automated librarian sorting your documents into categories like ‘legal contracts,’ ‘client PII,’ or ‘critical business files.’ This clarity empowers your organization to secure data intelligently and act with precision.
Dynamic Security Policies: Real-Time Defense in a Changing Landscape
The evolving value of data underscores the critical role of content analytics in ensuring security. A document’s significance can shift dramatically over time—for instance, an ordinary report might become sensitive when updated with personal or financial information. Such dynamic contexts demand equally agile security policies. This is where AI-powered content analytics plays a pivotal role. By continuously analyzing and categorizing data, it detects changes in content sensitivity and automatically adjusts security protocols. For example, when a report is modified to include customer credit card details, dynamic security policies—driven by content analytics—immediately spring into action. They might encrypt the file, limit user access, or trigger alerts for stakeholders. This proactive approach ensures not only compliance but also resilience against potential breaches. Content analytics enhances dynamic security policies by providing real-time insights into the data lifecycle. It evaluates patterns, flags anomalies, and assigns risk levels, enabling security measures to adapt instantly. This shifts organizations from a reactive stance to a preemptive one, addressing risks before they escalate.
Data Lineage: Following the Digital Footprint of Your Files
Every file tells a story, but in the world of unstructured data, those stories are often lost. Who accessed a document? What changes were made? Where did it go next? These questions are critical for compliance, security, and operational insights. Data lineage provides the answers, offering a detailed, timestamped trail of every interaction a file undergoes—edits, transfers, downloads, and views. It’s not just about transparency; it’s about control. For businesses facing stringent regulatory requirements, data lineage serves as an invaluable audit trail, ensuring accountability at every step. Data lineage turns your unstructured data into an open book, providing clarity and confidence.
DSPM and Unstructured Data Management: A Partnership for Proactive Protection
Security isn’t just about responding to breaches—it’s about preventing them. Data Security Posture Management (DSPM) adds a proactive layer of defense by continuously monitoring who is accessing your data and how. When paired with unstructured data management tools, DSPM becomes even more powerful. Picture this: a user downloads an unusually large number of sensitive files late at night. DSPM flags this as suspicious in real-time, alerting your team before any harm is done. Combined with unstructured data insights, this synergy provides a 360-degree view of your data landscape, spotting anomalies and blocking risks before they turn into crises. It’s more than a security measure—it’s a strategy for safeguarding your organization’s future.
Data Lifecycle Management: From Chaos to Compliance
Data, like anything else, has a lifecycle. Yet, unstructured data often lingers far beyond its usefulness, creating clutter, driving up storage costs, and increasing exposure to risks. Lifecycle management automates the process of managing this data, ensuring it’s retained only for as long as needed and securely disposed of when it’s no longer relevant. Compliance is a key driver here—regulations often require strict adherence to retention schedules. For instance, sensitive financial data may need to be archived for seven years, but anything beyond that could become a liability. Automated lifecycle management not only ensures compliance but also reduces the risk of breaches by minimizing the amount of obsolete data available to bad actors. It’s the ultimate cleanup strategy for organizations looking to maintain a lean, efficient, and secure data environment.
In a nutshell, managing unstructured data is more than just organizing files; it’s setting up the data ecosystem so that privacy and security happen automatically. From intelligent tagging and tracking to proactive threat detection, these tools create an environment where data is protected by design, not as an afterthought. If we want to secure our data for real, unstructured data management is where it all starts.
The Road Ahead
In today’s digital age, the challenges of managing unstructured data are escalating, driven by the growing complexity of data ecosystems and increasing regulatory demands. Data privacy and security are no longer optional; they are foundational to building trust and driving innovation. Yet, traditional methods often fall short in meeting the dynamic needs of organizations dealing with vast volumes of unstructured data.
This is where Zubin, Data Dynamics’ AI-powered self-service data management software, redefines the landscape. Zubin empowers organizations with unparalleled visibility and control over their data assets. Its robust capabilities include AI/ML-driven data observability, automated data classification, and advanced security orchestration, enabling businesses to understand, manage, and secure their unstructured data with precision. By offering tools for data lineage, policy-based governance, and root cause analysis, Zubin ensures compliance with evolving global regulations while reducing risks associated with data sprawl.
Zubin is not just a solution; it is a strategic ally in transforming data into a powerful asset. It provides the flexibility needed to navigate hybrid-cloud environments, optimize data mobility, and streamline lifecycle management, all while embedding privacy by design into every aspect of data handling. This approach fosters a secure, compliant, and sustainable data ecosystem that supports the ethical use of AI and the empowerment of data owners.
In a world where trust is built on the foundation of data integrity, Zubin enables organizations to proactively address challenges and unlock the full potential of their unstructured data. It positions enterprises to lead with confidence in an era defined by data-driven innovation and accountability. For more on how Zubin can revolutionize your data management strategy, visit www.datadynamicsinc.com or get in touch with us at www.datadynamicsinc.com/contact-us/.