- Data analytics is revolutionizing healthcare revenue cycle management (RCM), providing invaluable insights and optimizing revenue generation.
- Inefficient RCM processes can lead to 5% to 10% revenue loss, but data analytics helps reduce claim denials and identify revenue leakage points.
- Successful implementation of data analytics for RCM starts with understanding your data inside out.
In the fast-paced and ever-changing world of healthcare, financial stability, and operational efficiency have become absolutely crucial for healthcare organizations. The intricacies of revenue cycle management (RCM) are constantly evolving, pushing healthcare providers to seek out advanced technologies that can optimize their financial processes and boost revenue generation. Data analytics is stealing the spotlight as a total game-changer in this realm, offering invaluable insights and transforming how revenue is managed within the healthcare industry.
According to the American Medical Association (AMA), inefficient revenue cycle processes can lead to a staggering revenue loss of 5% to 10% for healthcare organizations. Yikes! That’s a lot of money left on the table. But here’s where data analytics swoops in to save the day! Data analytics involves collecting, interpreting, and using vast amounts of data to unveil patterns, trends, and actionable insights. With this powerful tool in their arsenal, healthcare organizations can navigate the complexities of RCM with unprecedented precision, agility, and foresight. From handling claims and analyzing reimbursements to pinpointing revenue leakage points, data analytics is revolutionizing how financial operations are carried out in the healthcare sector.
Intrigued yet? Well, hold on because we’re just getting started! In this article, we’ll dive headfirst into the world of data analytics for revenue cycle management in healthcare. We’ll explore the many ways data analytics is optimizing processes, boosting revenue streams, and driving operational excellence in the industry. By harnessing the incredible potential of data analytics, healthcare organizations can unlock new avenues for financial growth, streamline their operations, and, most importantly, enhance the quality of patient care. So, let’s journey together into the data-driven future of healthcare revenue management and witness the incredible transformations it brings!
What is RCM?
Do you know how healthcare providers deal with the financial side of patient care? It’s called revenue cycle management (RCM), and it’s a pretty comprehensive process. It involves things like patient registration, insurance verification, charge capture and coding, claims submission, payment posting, denial management, and reporting and analysis. Basically, it’s all about managing the money that comes in from patient services. The goal of RCM is to make billing and claims processes as smooth as possible, improving operational efficiency and thereby delivering a delightful patient care experience. Plus, it ensures that providers get paid appropriately for the services they provide.
But here’s the thing – RCM can be a bit of a puzzle. It’s complex, and sometimes it’s hard to tell if your RCM strategy is really doing its best to support your financial stability and enable the delivery of top-notch patient care. You want to make sure you’re not leaving money on the table and that your operations are running efficiently. Fortunately, there are ways to figure it out. By taking a closer look at your RCM processes and analyzing the data, you can get a clear picture of how things are working. You can identify areas for improvement, spot bottlenecks, and make informed decisions to optimize your revenue cycle. By streamlining billing, improving claims management, and keeping a close eye on the numbers, you can ensure that your organization is on the right track. It’s all about balancing financial stability, high-quality patient care, and operational efficiency.
Enhancing RCM with Data Analytics: 3 Key Benefits
Data analytics can have a transformative impact on revenue cycle management (RCM) strategy in healthcare. By harnessing the power of data, organizations can unlock a multitude of benefits. Here’s how:
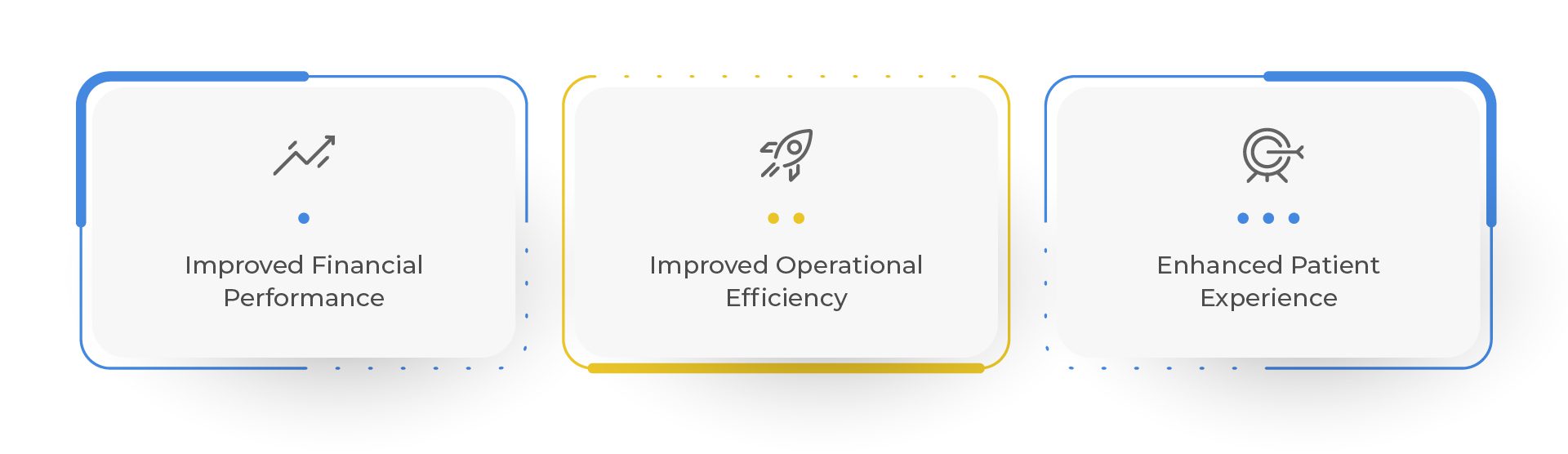
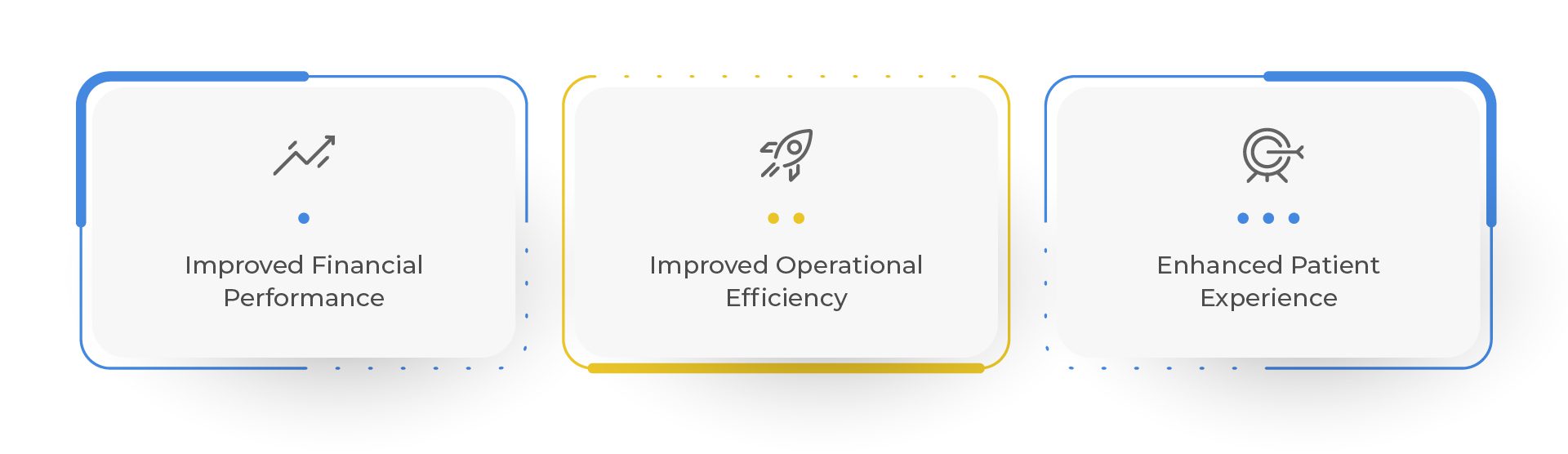
1. Improved Financial Performance: Data-driven approaches enable providers to develop more accurate revenue forecasts, enabling them to allocate resources more effectively and anticipate cash flow fluctuations. Furthermore, data analytics can identify patterns in claim denials, allowing for the development of strategies that reduce future denials and increase claim approvals. Organizations leveraging data analytics see an average improvement of 10-15% in clean claim rates and a reduction of 20-30% in claim denials.
- Claim Denial Reduction: Data analytics can analyze claim denials to identify patterns and root causes. By understanding the reasons behind denials, organizations can take proactive measures to minimize errors, improve claim accuracy, and reduce the number of denied or rejected claims. This leads to increased revenue capture and improved financial performance. Healthcare organizations utilizing data analytics experience a decrease in denial rates of up to 50%.
- Revenue Leakage Identification: Data analytics helps uncover revenue leakage points such as under-coding, missed charges, or incorrect pricing. By identifying and addressing these issues, healthcare organizations can capture previously lost revenue, contributing to improved financial performance. On average, there is a 1-5% revenue leakage due to coding errors that can be mitigated through data analytics.
- Revenue Forecasting: Leveraging historical data, predictive analytics can be applied to forecast future revenue. This enables organizations to make informed financial decisions, plan budgets effectively, and allocate resources efficiently. Data-driven revenue forecasting reduces forecasting errors by up to 20%.
2. Improved Operational Efficiency: In addition to improving financial performance, data analytics can contribute to operational efficiency as well. Providers can streamline processes by identifying inefficiencies in the revenue cycle, saving time and money. Data-driven RCM can also optimize resource allocation, ensuring that staff are deployed effectively, and administrative burdens are kept to a minimum. Organizations using data analytics experience a reduction of 20-30% in accounts receivable days.
- Workflow Optimization: Data analytics enables organizations to analyze operational workflows, identify bottlenecks, and streamline processes. By optimizing workflows, RCM teams can work more efficiently, reducing manual effort and increasing productivity.
- Performance Metrics Tracking: Data analytics allows organizations to track key performance indicators (KPIs) such as average collection time, days in accounts receivable, and first-pass claim acceptance rate. Monitoring these metrics helps identify areas for improvement, measure progress, and enhance operational efficiency.
- Resource Allocation: Data analytics can provide insights into resource utilization, allowing organizations to allocate staff and other resources effectively. This ensures that the right resources are available at the right time, reducing delays and optimizing productivity.
3. Enhanced Patient Experience: RCM strategies that leverage data can improve patient satisfaction and loyalty, thus contributing to long-term financial stability for healthcare organizations.
- Personalized Financial Assistance: Data analytics can analyze patient financial data to identify individuals who may require financial assistance. By understanding patients’ payment behaviors and financial situations, organizations can offer personalized payment plans or connect eligible patients with financial assistance programs. This improves the patient experience and increases the likelihood of timely payments.
- Streamlined Billing and Payment Processes: By analyzing data on billing and payment patterns, organizations can identify areas for improvement in the billing process, such as simplifying statements or offering convenient online payment options. Streamlining these processes reduces patient confusion, enhances satisfaction, and improves the overall patient experience.
Although the advantages of implementing data analytics in RCM are transformative, it’s crucial to acknowledge and tackle the accompanying challenges head-on to fully capitalize on the potential benefits. Let’s dive into some typical hurdles that arise when implementing data analytics in RCM.
Implementing Data Analytics in RCM – 6 Challenges. 6 Solutions
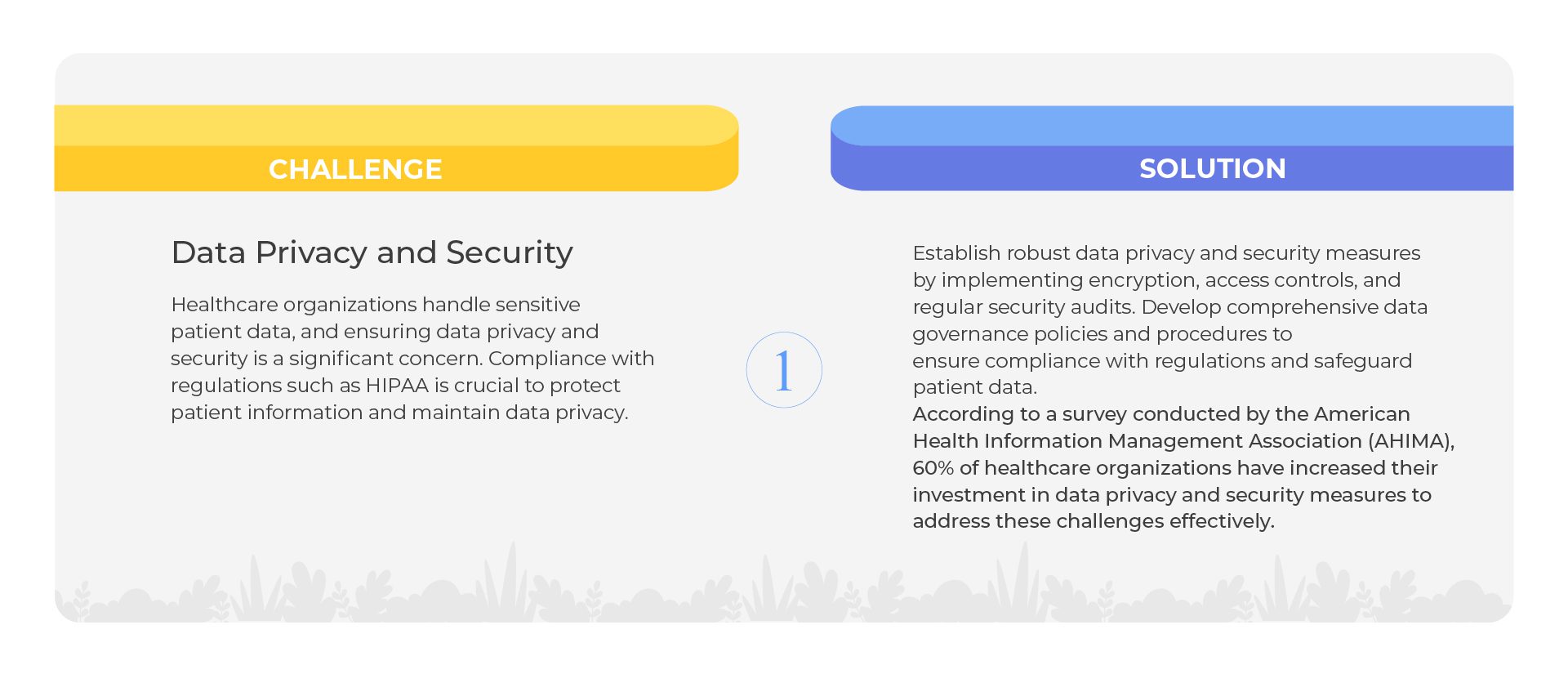
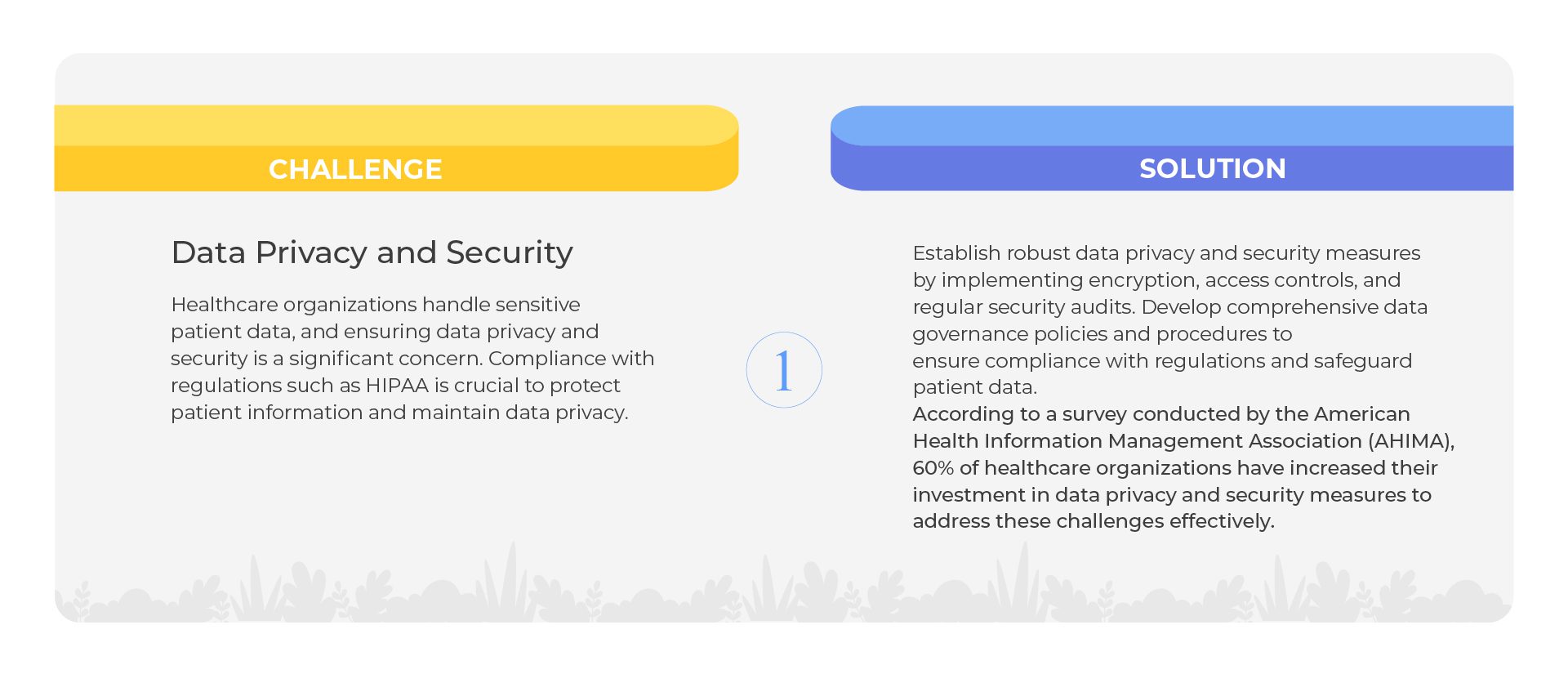
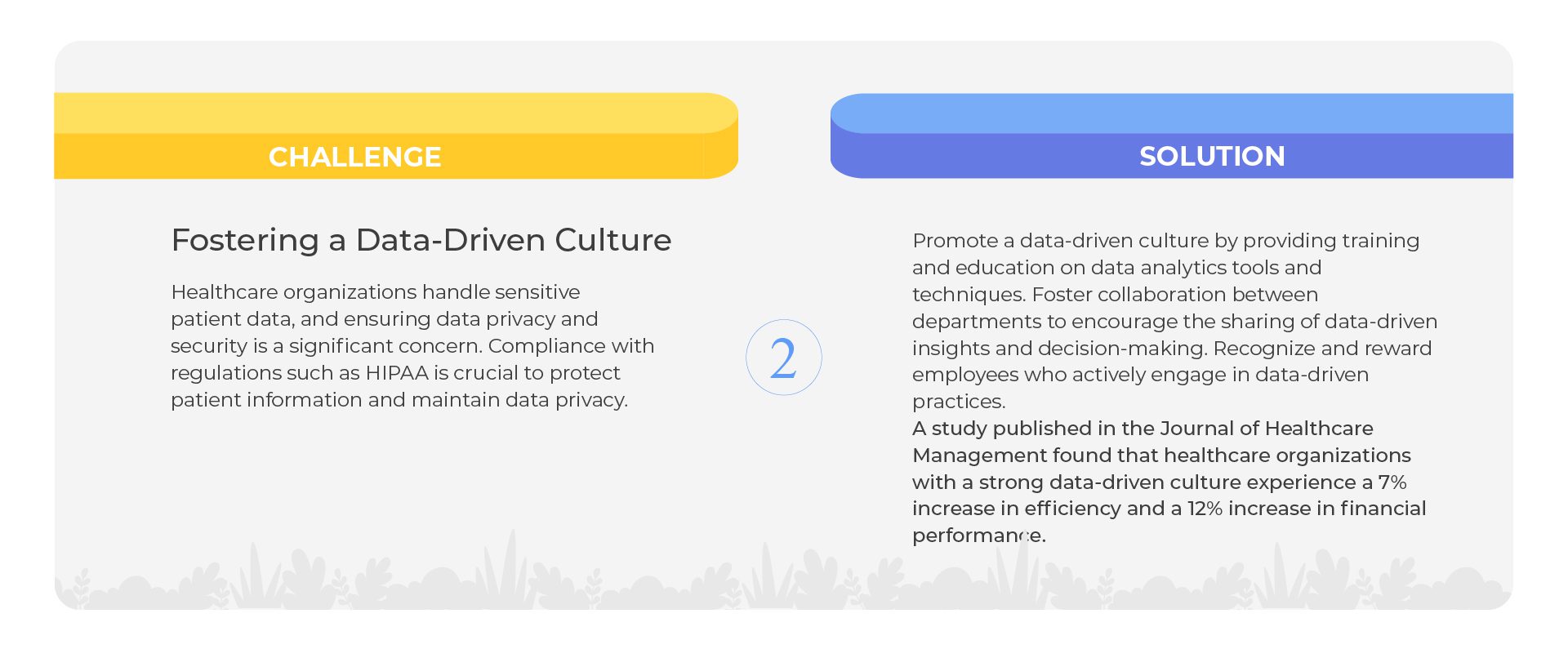
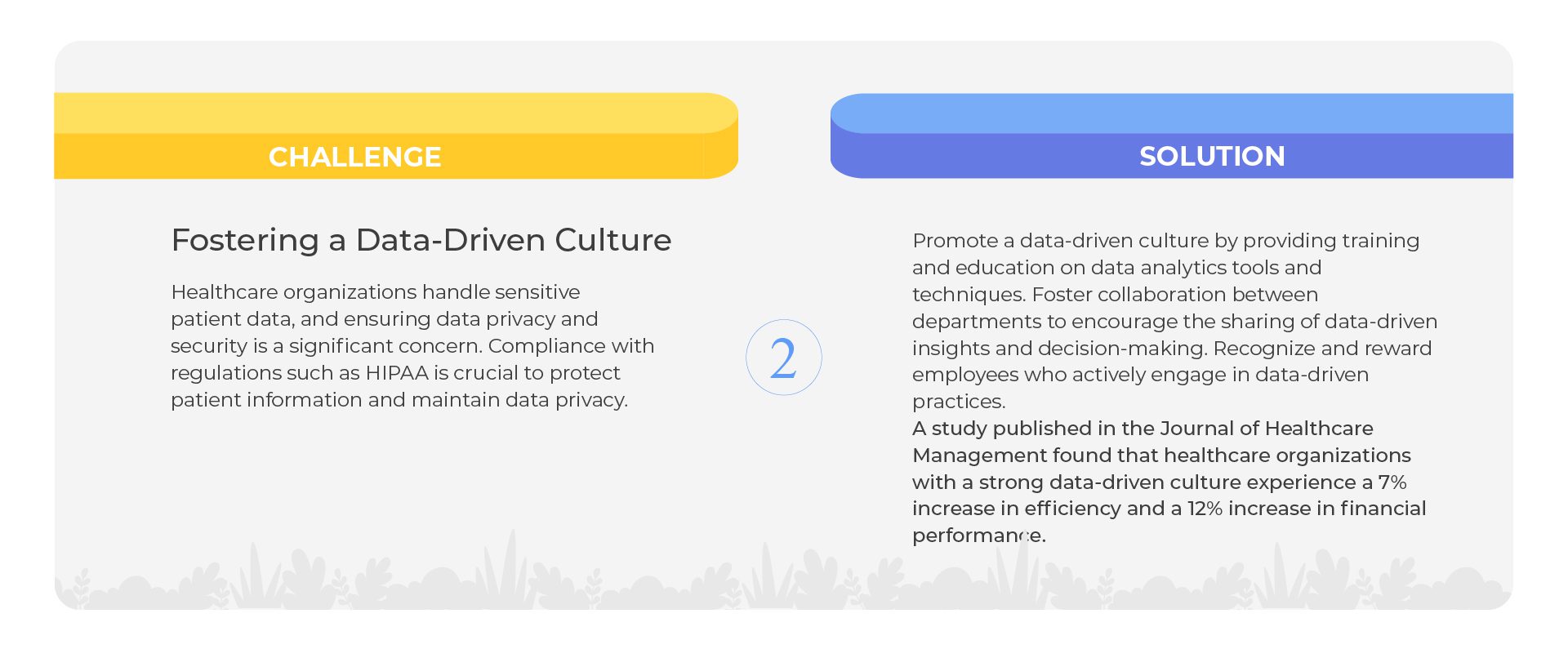
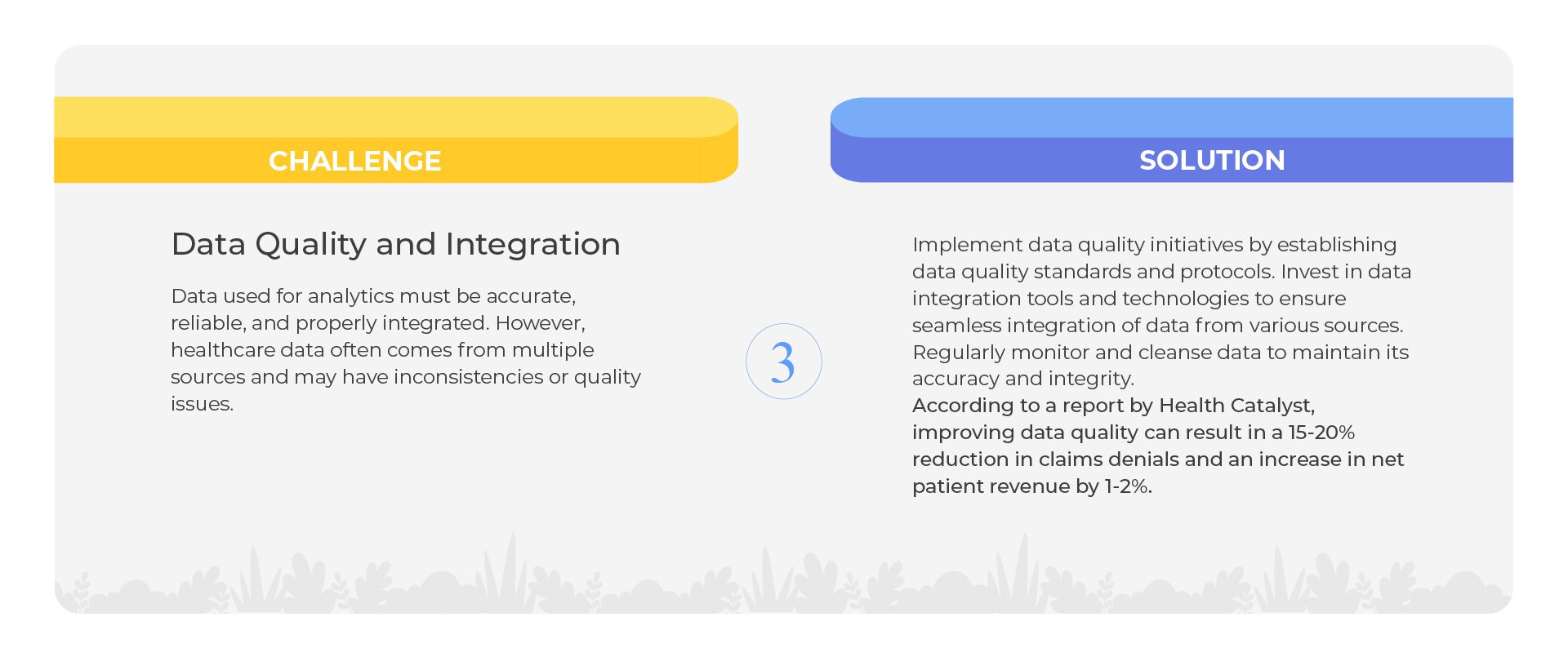
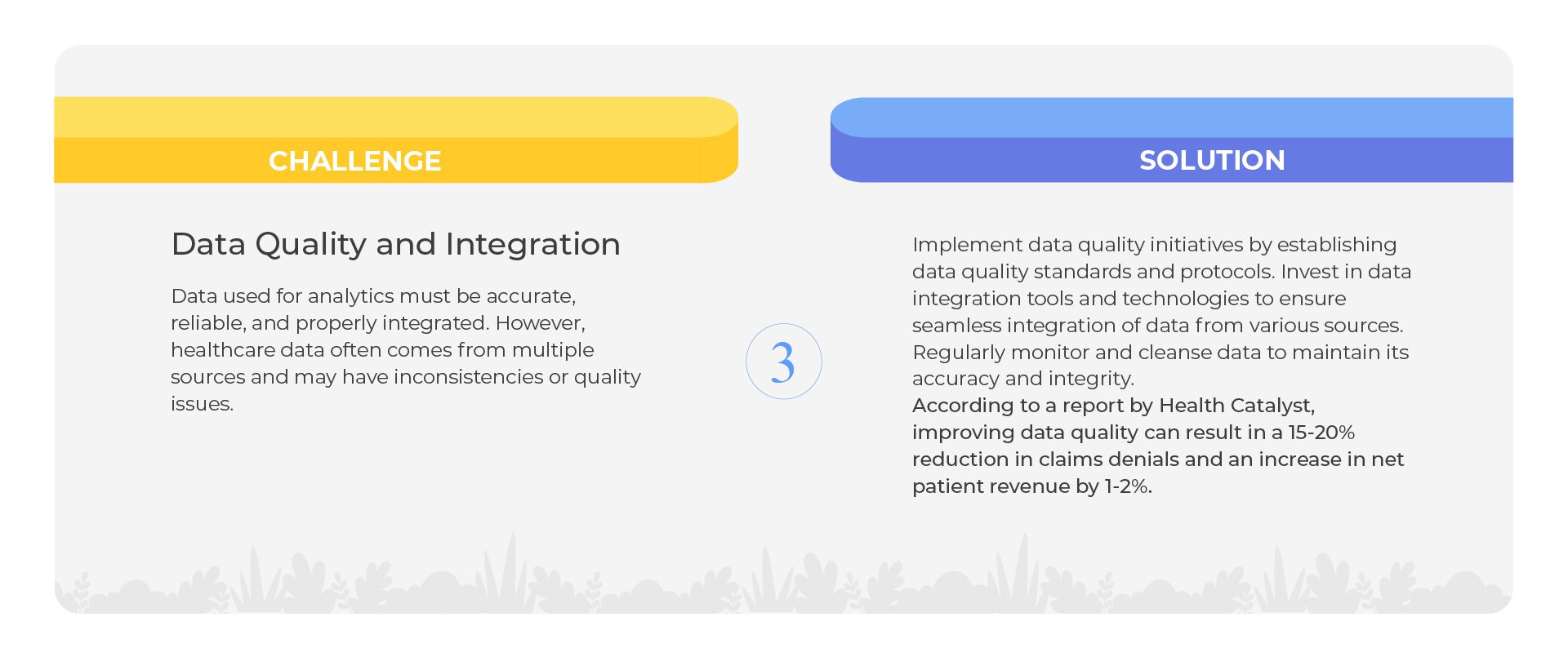
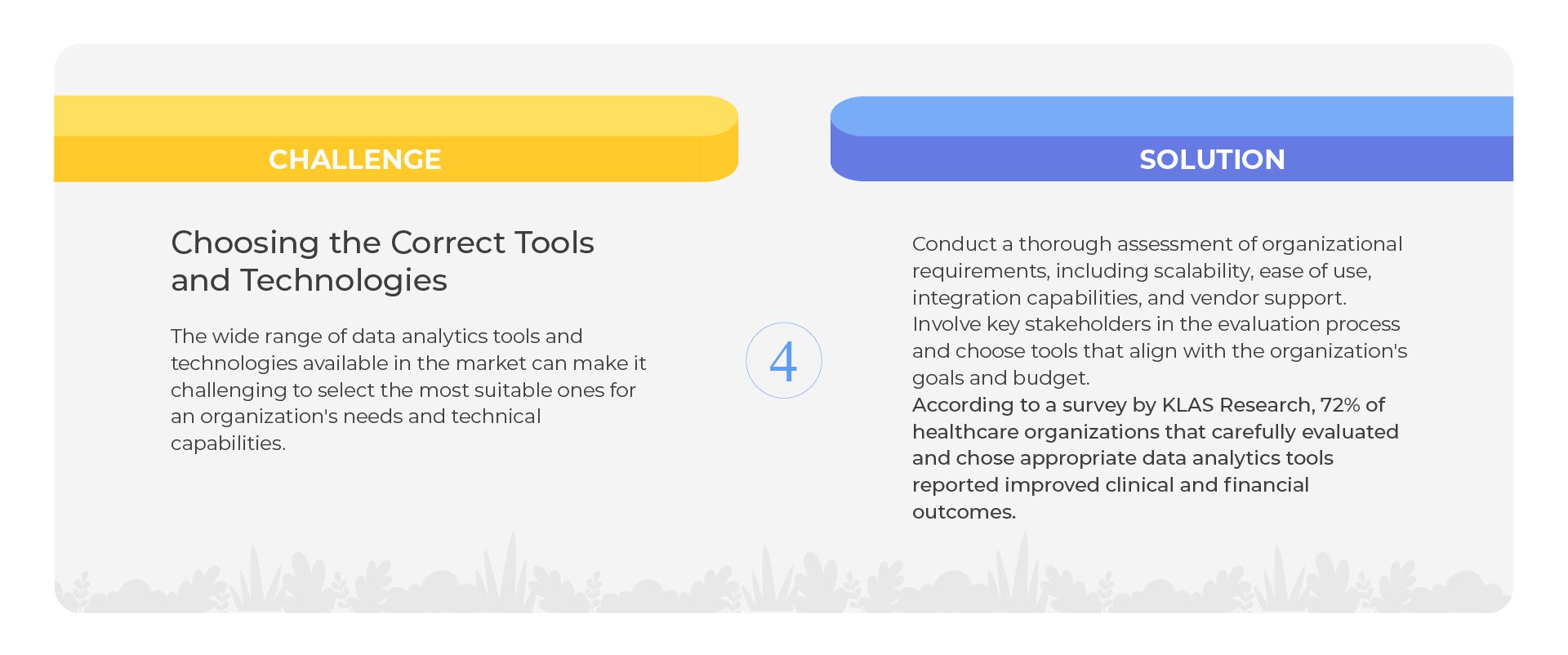
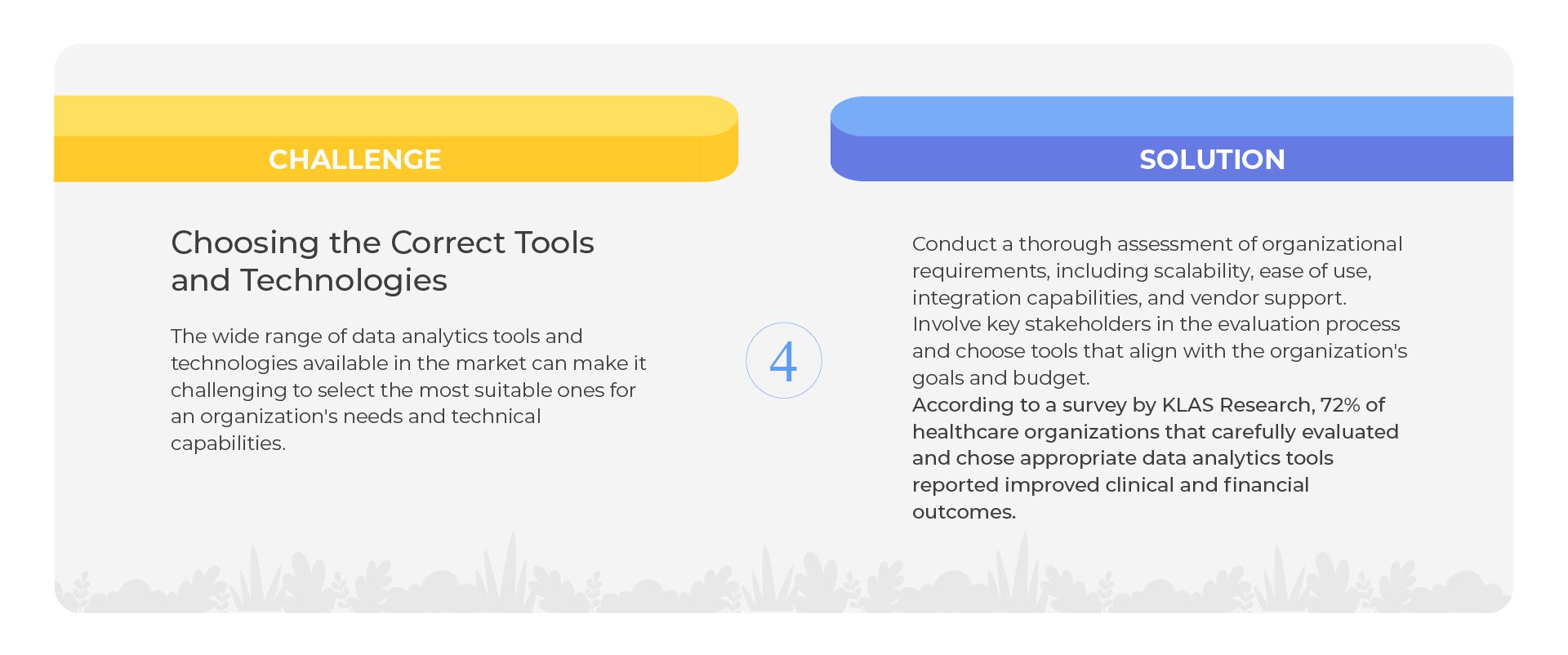
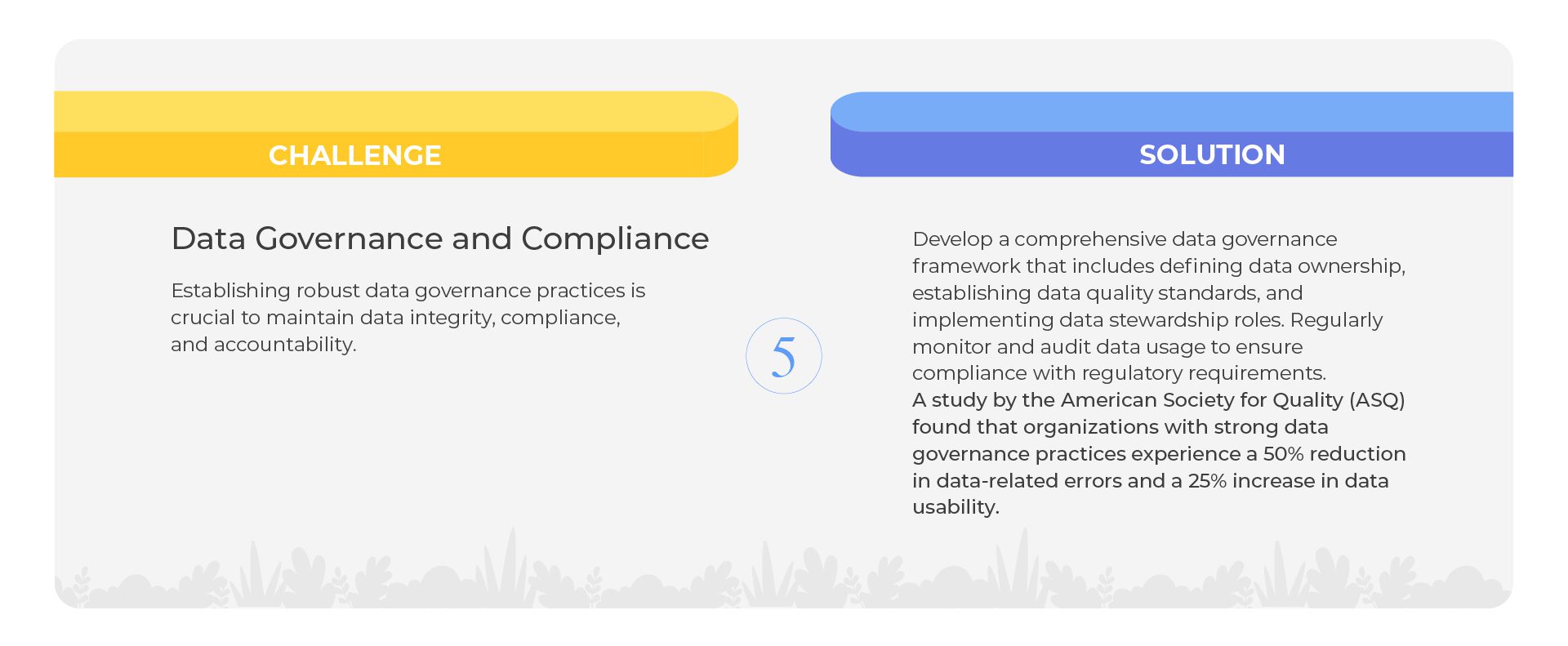
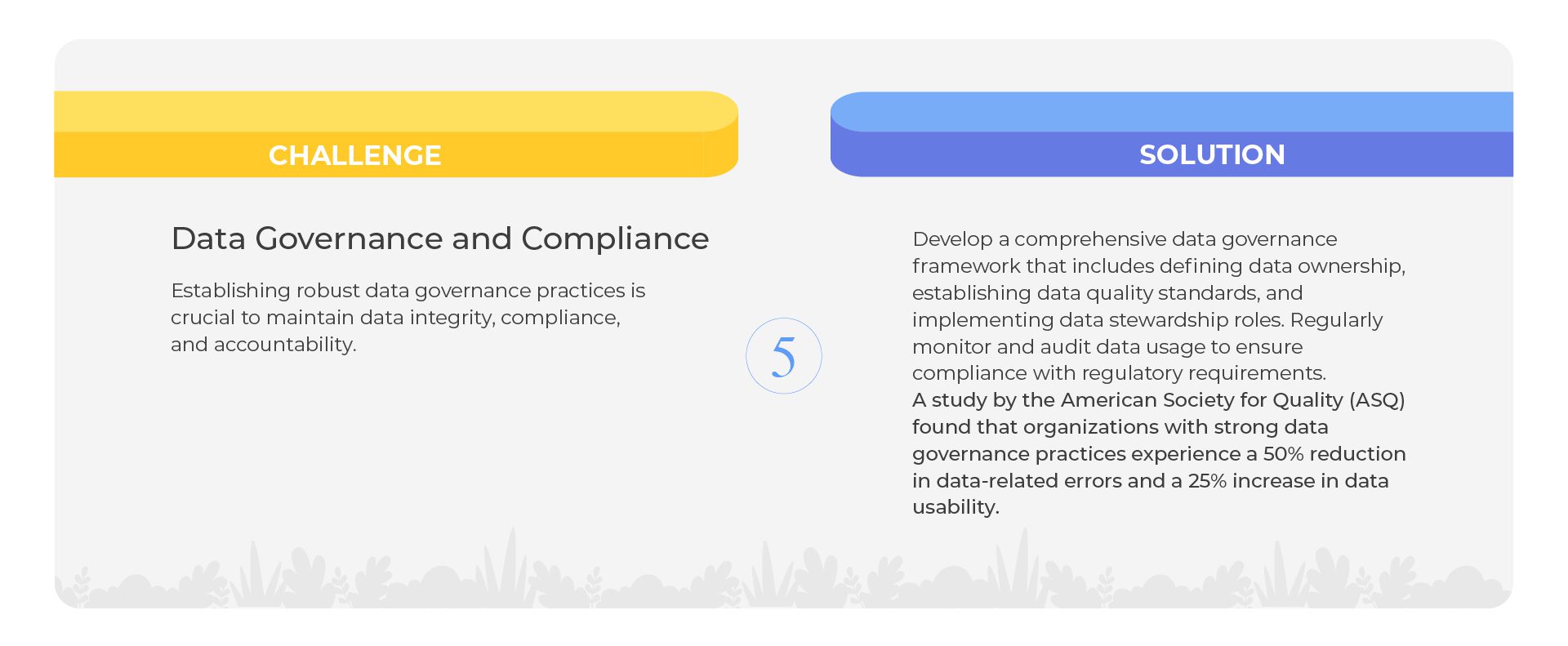
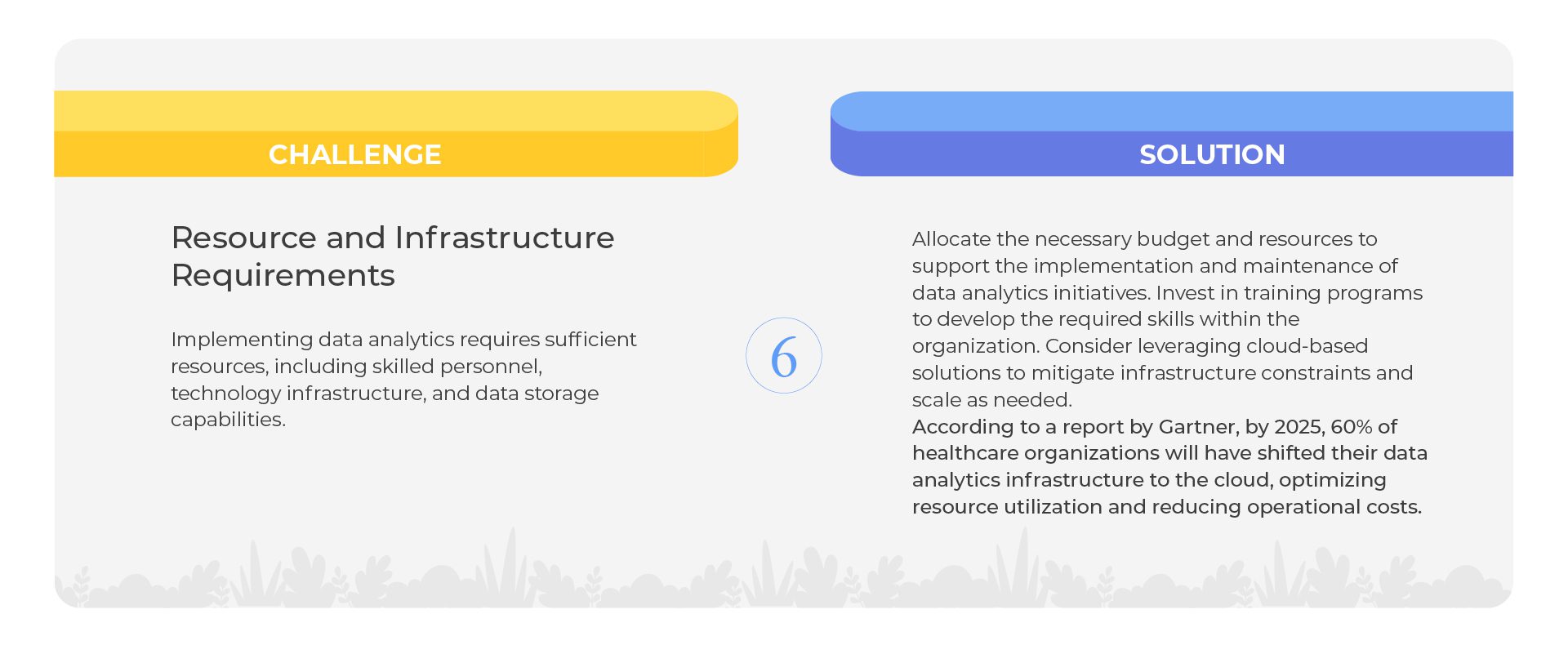
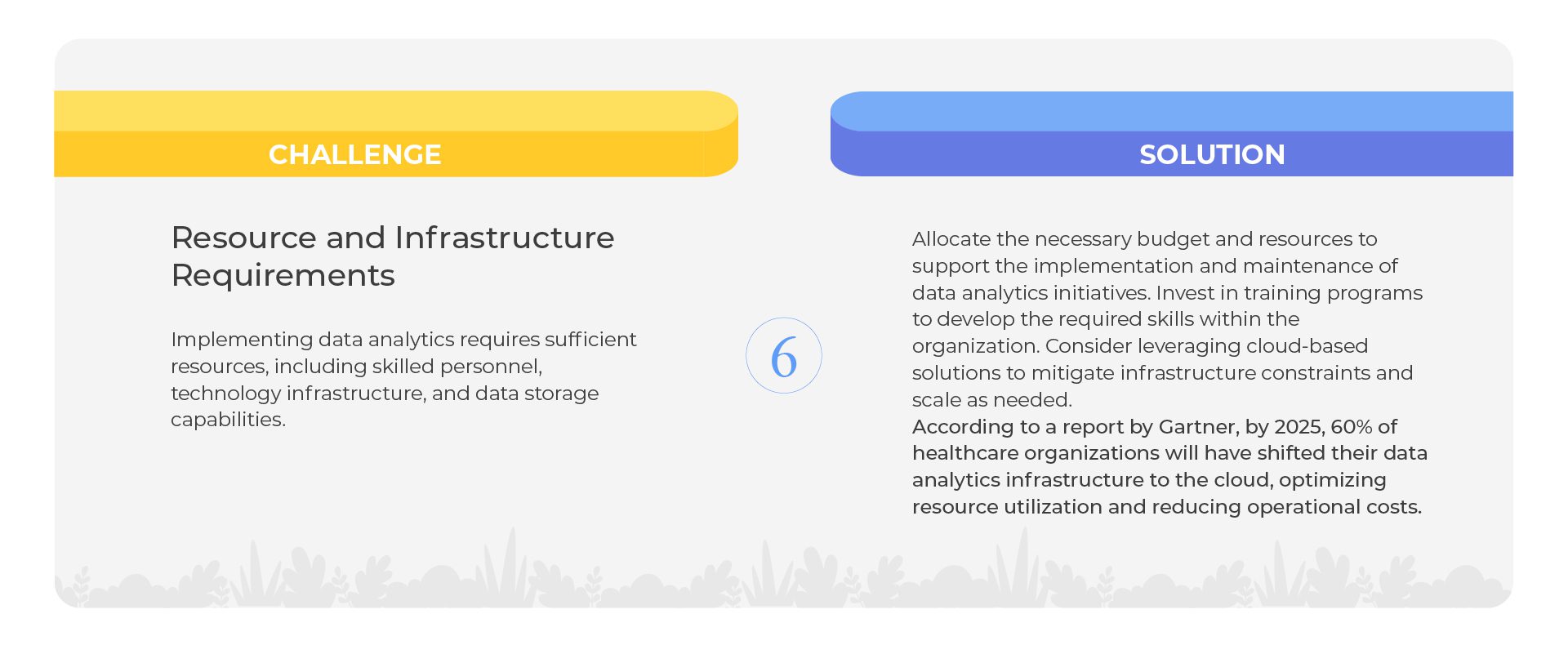
Here are some examples of healthcare organizations that have implemented data analytics for RCM and seen remarkable results:
- Cleveland Clinic: Cleveland Clinic, a renowned healthcare system, implemented data analytics to improve revenue cycle operations. By analyzing claim denials and reimbursement trends, they identified areas for improvement and implemented process changes to reduce denials, increase clean claim rates, and expedite reimbursement.
- Mayo Clinic: Mayo Clinic adopted data analytics to optimize its RCM strategy. They used predictive analytics to forecast revenue, identify potential revenue leakage points, and streamline billing processes. This resulted in improved financial performance and enhanced operational efficiency.
- Intermountain Healthcare: Intermountain Healthcare, a leading integrated delivery network, leveraged data analytics to reduce denials and improve collections. By analyzing claims data, they identified denial patterns, implemented corrective measures, and optimized their revenue cycle processes to enhance revenue capture.
- Mount Sinai Health System: Mount Sinai Health System implemented data analytics to streamline its revenue cycle operations. They utilized analytics to identify and address coding errors, improve charge capture processes, and optimize reimbursement workflows. This led to increased revenue and improved operational efficiency.
- Geisinger Health System: Geisinger Health System utilized data analytics to enhance its RCM processes. They leveraged predictive analytics to forecast revenue, identify opportunities for optimization, and proactively address claim denials. This resulted in improved financial performance and increased revenue capture.
These instances illustrate how healthcare organizations have effectively integrated data analytics into their RCM strategies, leading to improved financial performance, operational efficiency, and revenue optimization. However, there’s a challenge that must be addressed. To successfully implement data analytics for RCM, you must disentangle yourself from the web of unstructured data, which contributes to data deluge and data silos. This well-known challenge hinders analytics and, regrettably, accounts for 80% of enterprise data. Let’s explore how to overcome this obstacle.
Know your Data – The First Step to Implementing Data Analytics in RCM
The first and most critical step to implementing data analytics in Revenue Cycle Management (RCM) is to understand your data. Before diving into any data analysis or using sophisticated analytics tools, healthcare organizations must have a clear grasp of their data sources, data quality, and data architecture. Understanding your data lays the foundation for successful data analytics initiatives and ensures that you derive meaningful insights to optimize your RCM processes.
Here’s a closer look at why understanding your data is essential and how to approach it:
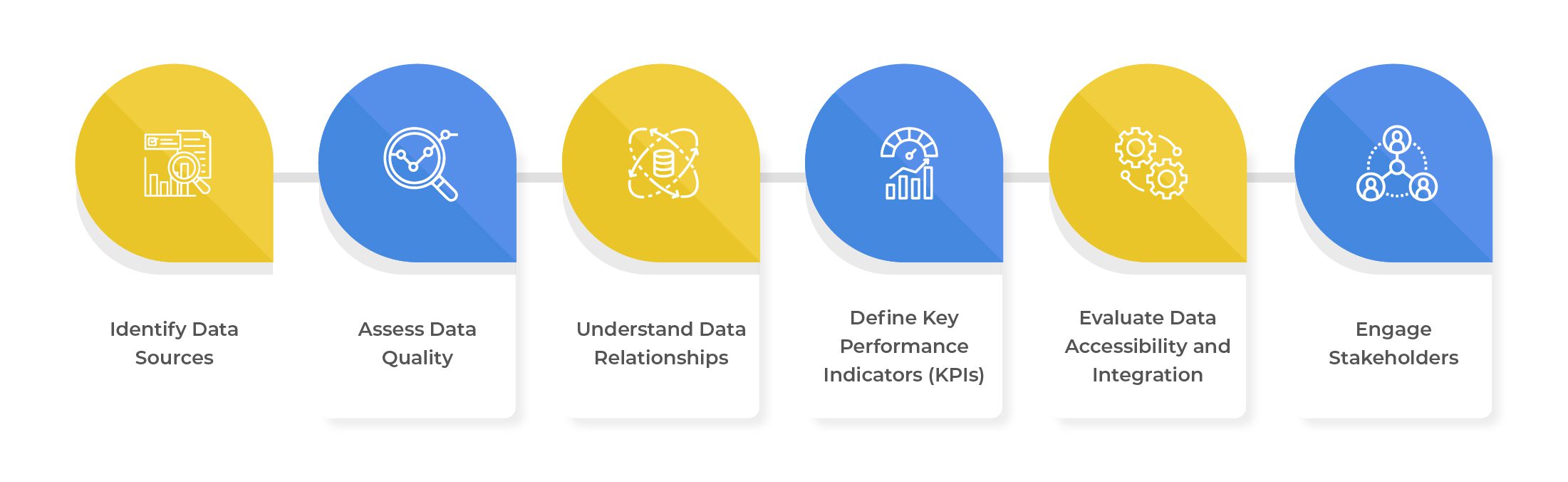
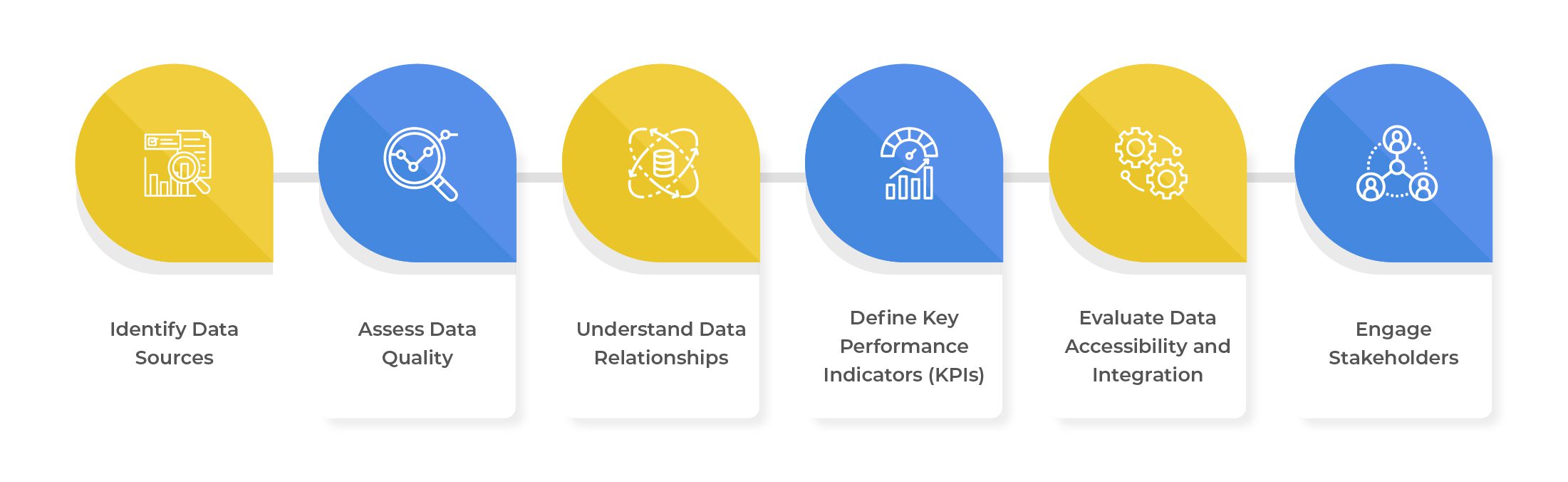
- Identify Data Sources: To implement data analytics successfully in Revenue Cycle Management (RCM), it is crucial to start by answering these questions – What data is being collected? Where does it come from? Where does it reside? Who accessed it last, and for what purpose? Who owns it and controls its use, analysis, storage, and disposal? Which data is ROT (redundant, obsolete, trivial) and can be safely purged? Which data is hot and which is cold, guiding storage optimization? How much orphan, aged, and duplicate data is lurking? How many open shares are out there? Which data can be archived, preserving longevity and simplifying retrieval? This involves identifying the various data sources contributing to the revenue cycle, such as electronic health records (EHRs), patient billing systems, claims data, financial reports, and other relevant sources. Analyzing metadata, including data tags, data lineage, and data definitions, aids in categorizing and organizing data sources, simplifying data management, and tracking throughout the RCM process.
- Assess Data Quality: Ensuring data quality is of paramount importance for effective data analytics. Evaluate the accuracy, completeness, and consistency of your data, as inaccurate or incomplete data can lead to erroneous conclusions and impede analytics initiatives. To complement structured data analysis, leverage content analytics techniques to extract insights from unstructured data like medical notes, billing comments, and patient feedback. This enriches the understanding of patient interactions and billing processes. Through data audits and assessments, identify any data quality issues and take steps to rectify them.
- Understand Data Relationships: Data in RCM often exhibits interconnectedness and dependencies on various factors. Employ context analytics to examine data within specific events or circumstances. For instance, context analytics can help understand the reasons behind claim denials by analyzing related factors like payer policies, coding guidelines, and patient demographics. Visualizing data relationships through data flow diagrams or entity-relationship models aids in comprehending the data’s structure and identifying crucial correlations.
- Define Key Performance Indicators (KPIs): Define key performance indicators (KPIs) aligned with your organization’s RCM goals to extract meaningful insights. These KPIs may include clean claim rates, denial rates, days in accounts receivable, and revenue capture rates. Well-defined KPIs guide data analytics efforts, providing a clear focus for analysis. Additionally, utilize content analytics to identify relevant patterns and trends in unstructured data, such as billing narratives or patient feedback, refining KPIs and obtaining a comprehensive understanding of RCM performance.
- Evaluate Data Accessibility and Integration: Ensure data accessibility and integration by providing the necessary access to data for analysis. As data may be distributed across various systems or databases, consider data integration tools and technologies to create a unified data repository for analysis. Leverage metadata analytics to monitor data access permissions and integration processes, guaranteeing data security and integrity throughout the analytics journey.
- Engage Stakeholders: Engage key stakeholders, such as revenue cycle managers, billing specialists, and data analysts, in the data understanding process. Collaborating with stakeholders offers valuable insights into RCM challenges and opportunities. Incorporating context analytics helps stakeholders understand the real-world implications of data analytics insights, enabling them to interpret findings effectively and make informed decisions based on the analysis.
The Data Dynamics Advantage
Data Dynamics stands at the forefront as a leading provider of an enterprise unified data management platform, presenting a comprehensive all-in-one software solution for enterprises to maximize the value of their unstructured and voluminous data securely and under governance. Employing a four-module approach encompassing Analytics, Security, Compliance, and Mobility, Data Dynamics aids businesses in navigating the intricacies of unstructured data and facilitating effective data analytics for Revenue Cycle Management (RCM):
- Data Integration and Aggregation: The platform ingests and consolidates various unstructured data sources, creating a centralization platform for enterprise data and simplifies data access & analysis for RCM.
- Data Cleaning and Transformation: Unstructured data often contains inconsistencies and noise. Data Dynamics offers capabilities to clean, preprocess, and transform the data, ensuring its quality and compatibility with the analytics process.
- Data Cataloging and Metadata Management: Data Dynamics creates a data catalog enriched with relevant metadata for unstructured data, facilitating efficient search, understanding, and locating of data needed for RCM analysis.
- Advanced Analytics and AI: Data Dynamics provides advanced analytics tools and AI capabilities, including natural language processing (NLP) and content analytics, and other techniques to gain valuable insights from various data types.
- Data Governance and Compliance: The Data Dynamics platform is designed to empower organizations with comprehensive insights into their PII data landscape and enable effective risk mitigation to ensure data security and adherence to regulations like HIPAA, thus maintaining data privacy and compliance for RCM.
- Visualization and Reporting: The platform offers data visualization and reporting tools to present insights in a meaningful and easily understandable manner for RCM stakeholders.
- Data-Driven Decision Making: Leveraging insights from data analytics, Data Dynamics empowers companies to make data-driven decisions, enhancing the effectiveness and efficiency of revenue cycle management.
To learn more about Data Dynamics, please visit – www.datadynamicsinc.com or contact us at solutions@datdyn.com I (713)-491-4298.